By Evan Charney, Duke Institute for Brain Science, Duke University
One of the hopes and promises of the Human Genome Sequencing Project was that it would revolutionize the understanding, diagnosis, and treatment of most human disorders. It would do this by uncovering the supposed “genetic bases” of human behavior. With a few exceptions, however, the search for common gene variants -“polymorphisms” – associated with common diseases has borne little fruit. And when such associations have been found the polymorphisms seem to have little predictive value and do little to advance our understanding of the causes of disease. In a 2012 study, for example, researchers found that incorporating genetic information did not improve doctors’ ability to predict disease risk for breast cancer, Type 2 diabetes, and rheumatoid arthritis [1].
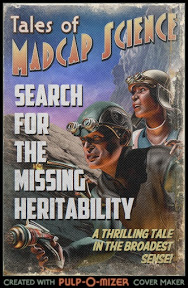
And to date, not a single polymorphism has been reliably associated with any psychiatric disorders nor any aspect of human behavior within the “normal” range (e.g., differences in “intelligence”).
To some researchers this state of affairs has given rise to a conundrum known as the “problem of missing heritability.” If traits such as intelligence are reported to be 50% heritable, goes the theory, why have no genes associated with intelligence been identified? One possible solution to the problem of missing heritability is that the heritability estimates are wrong. Another proposal is that hundreds or even thousands of genes are involved, each gene of such small effect that it cannot be identified by the standard genome-wide association study (GWAS). These problems have spurred the development of a new methodology for identifying gene variants in human populations called genome-wide complex trait analysis (GCTA).
Enter Genome-wide complex trait analysis (GCTA)
The first results of a GCTA study were published in 2010 [2]. Since then its use has rapidly expanded with the results of GCTA studies on everything from obesity to intelligence to autism regularly appearing in prestigious science journals [3-17]. Like a typical GWAS, GCTA involves scanning hundreds of thousands of polymorphisms (specifically, a common form of gene variant known as a single nucleotide polymorphism [SNP]) of thousands of persons. But instead of trying to identify individual polymorphisms more common among those who share a given trait, the goal is to determine whether or not this trait similarity can be associated with a large number of (unidentified) polymorphisms. In other words, an estimate is generated as to how much of the genetic variance (i.e., heritability) of a trait can be accounted for by shared SNPs. These heritability estimates are termed “SNP-based” and differ from standard heritability estimates that rely upon assumptions of genetic relatedness, such as twin or family studies.
For example, the much used twin study methodology is based upon the assumption that monozygotic (MZ) twins share 100% of their inherited genes, as compared to “fraternal” or dizygotic (DZ) twins who share on average 50% of their inherited genes. If MZ twins show greater concordance for a trait of interest than DZ twins, this greater concordance is ascribed to greater genetic concordance, with the presumed genetic relationship of 1 to .5 serving as the basis of the heritability estimate. By contrast, GCTA does not rely upon genetic relatedness. In fact, it is critical to GCTA that those who are studied be unrelated.
The twin study methodology has long been critiqued as being based upon a number of faulty assumptions, in particular the assumption that the environments (pre and postnatal) of MZ twins are not more alike than DZ twins. Were the environments of MZ twins more alike than those of DZ twins (as numerous studies have indicated), trait similarities ascribed to the greater genetic similarity of MZ twins might in fact be due to greater environmental similarity, significantly inflating heritability estimates. Thus far, GCTA studies appear to have proven critics of the twin study methodology right, yielding significantly lower heritability estimates (e.g., an estimation of “callous-unemotional” behavior based on the twin study methodology yielded a heritability estimate of 64%, as compared to a GCTA that yielded a heritability estimate of 7% [9]). Long-time defenders of the accuracy of twin studies can now be found speculating, in light of GCTA findings, that “the estimates of … heritability from twin and family studies are biased upwards, for example, by not properly accounting for… (common) environmental factors” [7]. GCTA studies, however, just like their twin study predecessors, suffer from serious methodological problems that call into doubt the legitimacy of their findings. They, too, are likely to generate spurious associations and faulty estimates of genetic contributions to variation in traits.
GCTA studies are highly vulnerable to confounding by population stratification
Genetic studies (by whatever method) that have so far purported to identify SNPs associated with one or another trait have more often than not been false positives [18-20]. A prime cause of this has been the failure of researchers to take adequately into account population stratification. Population stratification refers to the fact that frequencies of polymorphisms can differ in different populations and subpopulations (ethnic or geographical) due to unique ancestral patterns of migration, mating practices, and reproductive expansions and contractions. Nearly all outbred (i.e., nonfamilial) populations exhibit population stratification, including populations deemed relatively homogenous (e.g., among Icelanders). One well-known example of a false association between a polymorphism and a trait was the link between the dopamine receptor gene DRD2 and alcoholism. Initial studies suggested a strong association, but subsequent investigations found none when more effective controls for population stratification were imposed. In retrospect, it is clear why this initial result was vulnerable to confounding due to population stratification: DRD2 alleles vary widely by ethnic ancestry, and ethnic differences in alcoholism rates are pronounced.
Recall that GCTA studies are supposed to involve unrelated persons. From the standpoint of population genetics, however, relatedness is not simply a matter of being someone’s second cousin. While the designers of the GCTA method are aware of the problem posed by population stratification and attempt to correct for it, there is growing evidence that the techniques they have employed are wholly inadequate and that GCTA itself is particularly vulnerable to confounding due to population stratification [21-23].
Consider a recent GCTA study by Plomin et al., who reported a SNP-based heritability estimate of 35% for “general cognitive ability” among UK 12 year olds (as compared to a twin heritability estimate of 46%) [8]. According to the Wellcome Trust “genetic map of Britain,” striking patterns of genetic clustering (i.e. population stratification) exist within different geographic regions of the UK, including distinct genetic clusterings comprised of the residents of the South, South-East and Midlands of England; Cumbria, Northumberland and the Scottish borders; Lancashire and Yorkshire; Cornwall; Devon; South Wales; the Welsh borders; Anglesey in North Wales; Scotland and Ireland; and the Orkney Islands [8]. Now consider the title of a study from the University and College Union: “Location, Location, Location – the widening education gap in Britain and how where you live determines your chances” [9]. This state of affairs (not at all unique to the UK), combined with widespread geographic population stratification, is fertile ground for spurious heritability estimates.
Further problems of GCTA
While I have focused on population stratification, there are at least two other things to note about GCTA studies. First, GCTA assumes “additive genetic variance,” i.e., that each polymorphism contributes a tiny amount to heritability and that the “effects” of all the polymorphisms can simply be added together. This ignores widespread evidence that genes influence the effects of other genes in highly complex, non-additive ways (“G x G” interactions), and that the environment influences the manner in which genes are transcribed in equally complex ways (“G x E” interactions). Second, all GCTA estimates are derived from looking only at SNPs, but SNPs are only one form of genetic polymorphism. There are numerous other kinds of prevalent genetic variations, including copy number variations, multiple copies of segments of genes, whole genes, and even whole chromosomes. There is no rational scientific reason to assume that SNPs are the only relevant, or even the “most important” form of genetic variation (other than the fact that SNP data is easiest to obtain).
The simplistic model of additive genetic variance upon which GCTA relies (and which assumes no epistasis and no gene x environment interactions) is out of touch with current understanding of the complex, multifactorial nature of most human traits that have a genetic component. Consider Type I diabetes (T1D). Fewer than 10% of individuals who possess gene variants associated with T1D progress to the clinical disease; <40% of MZ twins are concordant for T1D; there is a more than a 10-fold difference in the disease incidence among Caucasians living in Europe; there has been a several-fold increase in the incidence over the last 50 years; and migration studies indicate that the disease incidence has increased in population groups who have moved from a low-incidence to a high-incidence region. What this indicates is not the presence of hundreds or thousands of hidden polymorphisms, but a complex interaction between genes, the developmental programming of the immune system, and an environmental “diabetogenic” trigger:
The identification of exogenous factors triggering and driving β-cell destruction [which results in the clinical disease] offers a potential means for intervention aimed at the prevention of T1D. Therefore, it is important to pursue studies on the role of environmental factors in the pathogenesis of this disease. Environmental modification is likely to offer the most powerful strategy for effective prevention of T1D, because such an approach can target the whole population or at least that proportion of the population carrying increased genetic disease susceptibility; therefore, preventing both sporadic and familial T1D, if successful [24, p. 13].
Or consider the effects of developmental stress on brain development and behavior. Monkeys generated from stressed mothers show significantly reduced hippocampal neurogenesis and significantly reduced hippocampal volume with corresponding cognitive and behavioral effects. Likewise in humans, prenatal stress has been associated with a wide array of adverse developmental cognitive and behavioral outcomes [25].
What these examples show is that if we want to understand human traits that have a genetic component, we must turn away from an excessive and offtimes exclusive focus upon genetic polymorphisms and take a more holistic approach, one in which disease and health are seen as attributes of plastic, adaptive organisms functioning within particular environments.
Advocates of GCTA, however, tell us that in order to find the multitude of polymorphisms of tiny effect underlying heritability estimates we must undertake ever larger studies involving hundreds of thousands of persons. These polymorphisms of tiny effect, however, are so many ghosts and the search for them is the last gasp of a failed paradigm. Do we really want to squander our time and resources chasing ghosts?
1. Aschard, H., et al., Inclusion of gene-gene and gene-environment interactions unlikely to dramatically improve risk prediction for complex diseases. Am J Hum Genet, 2012. 90(6): p. 962-72.
2. Yang, J., et al., Common SNPs explain a large proportion of the heritability for human height. Nat Genet, 2010. 42(7): p. 565-9.
3. Yang, L., et al., Polygenic transmission and complex neuro developmental network for attention deficit hyperactivity disorder: genome-wide association study of both common and rare variants. Am J Med Genet B Neuropsychiatr Genet, 2013. 162B(5): p. 419-30.
4. Rietveld, C.A., et al., Molecular genetics and subjective well-being. Proc Natl Acad Sci U S A, 2013. 110(24): p. 9692-7.
5. Llewellyn, C.H., et al., Finding the missing heritability in pediatric obesity: the contribution of genome-wide complex trait analysis. Int J Obes (Lond), 2013.
6. Keller, M.F., et al., Using genome-wide complex trait analysis to quantify ‘missing heritability’ in Parkinson’s disease. Hum Mol Genet, 2012. 21(22): p. 4996-5009.
7. Vinkhuyzen, A.A., et al., Common SNPs explain some of the variation in the personality dimensions of neuroticism and extraversion. Transl Psychiatry, 2012. 2: p. e102.
8. Plomin, R., et al., Common DNA markers can account for more than half of the genetic influence on cognitive abilities. Psychol Sci, 2013. 24(4): p. 562-8.
9. Viding, E., et al., Genetics of callous-unemotional behavior in children. PLoS One, 2013. 8(7): p. e65789.
10. Vrieze, S.I., et al., Three mutually informative ways to understand the genetic relationships among behavioral disinhibition, alcohol use, drug use, nicotine use/dependence, and their co-occurrence: twin biometry, GCTA, and genome-wide scoring. Behav Genet, 2013. 43(2): p. 97-107.
11. Power, R.A., et al., Estimating the heritability of reporting stressful life events captured by common genetic variants. Psychol Med, 2013. 43(9): p. 1965-71.
12. Trzaskowski, M., et al., First Genome-Wide Association Study on Anxiety-Related Behaviours in Childhood. PLoS ONE, 2013. 8(4): p. e58676.
13. Speed, D., et al., Improved Heritability Estimation from Genome-wide SNPs. American journal of human genetics, 2012. 91(6): p. 1011-1021.
14. Yang, J., et al., Ubiquitous polygenicity of human complex traits: genome-wide analysis of 49 traits in Koreans. PLoS Genet, 2013. 9(3): p. e1003355.
15. Watson, C.T., et al., Estimating the proportion of variation in susceptibility to multiple sclerosis captured by common SNPs. Sci Rep, 2012. 2: p. 770.
16. Klei, L., et al., Common genetic variants, acting additively, are a major source of risk for autism. Mol Autism, 2012. 3(1): p. 9.
17. Lee, S.H., et al., Estimating missing heritability for disease from genome-wide association studies. Am J Hum Genet, 2011. 88(3): p. 294-305.
18. Bosker, F.J., et al., Poor replication of candidate genes for major depressive disorder using genome-wide association data. Molecular Psychiatry, 2011. 16(5): p. 516-32.
19. Chabris, C.F., et al., Most reported genetic associations with general intelligence are probably false positives. Psychol Sci, 2012. 23(11): p. 1314-23.
20. Ioannidis, J.P., Non-replication and inconsistency in the genome-wide association setting. Hum Hered, 2007. 64(4): p. 203-13.
21. Browning, S.R. and B.L. Browning, Population structure can inflate SNP-based heritability estimates. Am J Hum Genet, 2011. 89(1): p. 191-3; author reply 193-5.
22. Janss, L., et al., Inferences from genomic models in stratified populations. Genetics, 2012. 192(2): p. 693-704.
23. Browning, S.R. and B.L. Browning, Identity-by-descent-based heritability analysis in the Northern Finland Birth Cohort. Hum Genet, 2013. 132(2): p. 129-38.
24. Knip, M. and O. Simell, Environmental triggers of type 1 diabetes. Cold Spring Harb Perspect Med, 2012. 2(7): p. a007690.
25. Coe, C. L., Kramer, M., Czéh, B., Gould, E., Reeves, A. J., Kirschbaum, C. & Fuchs, E. (2003) Prenatal stress diminishes neurogenesis in the dentate gyrus of juvenile rhesus monkeys. Biological Psychiatry 54(10):1025–34
If this article was useful to you please consider sharing it with your networks.
I agree with what is said here generally, but I don’t think it will make the missing heritability (Mh) ‘problem’ go away. There are too many people who for many different reasons believe that more sophisticated or greater sampling, or more extensive sequencing and analysis, and larger studies, paired with animal models, will eventually account for Mh. Whether this is a correct belief or as much a rationale for funding continual increases in study scale, is debatable.
Rare variants reflect one ‘out’ that is often invoked, and they certainly require large studies of one sort or another. The question here is whether enumerating rare variants and demonstrating their causal role (if it can actually be done) will do much, especially since most rare variants will be like their more common known ones, and have very small individual effects.
Another strategy is to blame the mH on interactions. Huge studies or very clever designs may identify such interactions and evaluate their import, perhaps at least generically if not by enumeration.
So the problem will, I predict, persist. That doesn’t mean the claims about how to find mH are justified.
Failed paradigms have a way of slouching on from beyond the grave after they’ve been declared dead, especially if they have become lucrative, prestigious, and elaborate industries supporting many livelihoods and paying the mortgages on many yachts. SNP-chasing, in particular in “psychiatric” behavioral syndromes, tends to exemplify the Festinger phenomenon, in which the more evidence that is adduced against a superstitious belief, the stronger the belief becomes. Festinger (who gave us the term “cognitive dissonance”) discovered that when practitioners of Voodoo were confronted with falsifying evidence, their belief became even stronger. It went something like this: If a skeptic shows me that sticking pins in the chest of a doll does not cause my enemy to have a heart attack, it is because Voodoo predicts that deceiving devils will try to persuade me against Voodoo. The skeptic is obviously the deceiving devil predicted by Voodoo, and hence, Voodoo is true. Thank you, Mr. Skeptic, because by coming to Haiti and trying to prove that Voodoo is false, you have proved that it is even truer. In the case of SNP hunters in human behavioral genetics, when negative evidence accumulates (such as nonreplicated, nonspecific, or contradictory findings), it is interpreted as proof that the assumed deleterious SNP-associated polygenesis underlying a target condition must be even more complex and elusive. This of course requires larger samples, finer grained sequencing – and more funding. The Festinger phenomenon can be interpreted cognitively as a homeostatic response to an external stress. In this case, a certain idea (“Voodoo is false”) causes anxiety in the Voodoo believer, who then generates a perfectly fitting “antibody idea” to the antigenic idea that binds itself to the antigen and neutralizes it. From the standpoint of molecular psychobiology, the threatening idea can be considered to lower the tonic endorphin level (creating anxiety, anger, unhappiness) associated with an intracerebral cognitive autoaddiction. Then Festingerization provides a junkie’s “fix”, restoring the previous equilibrium, but now at a higher daily dose of true belief. Or, heretics can simply be burned at the stake, end of problem.
In the GCTA approach discussed by Charney, the variance estimate is derived from the average genome-wide similarity between all pairs of individuals determined using all SNPs. This is “genetic profiling” on steroids. In 2012 some prominent SNP hunters used the GCTA approach on a large case control study of schizophrenics of European descent to survey the SNP landscape, or genetic architecture, of schizophrenia (SZ). (“Estimating the proportion of variation in susceptibility to schizophrenia captured by common SNPs”. Lee et al, Nature Genetics 44(3):247-250) The abstract illustrates the kinds of boilerplate problems discussed by Charney:
Schizophrenia is a complex disorder caused by both genetic
and environmental factors. Using 9,087 affected individuals,
12,171 controls and 915,354 imputed SNPs from the
Schizophrenia Psychiatric Genome-Wide Association Study
(GWAS) Consortium (PGC-SCZ), we estimate that 23%
(s.e. = 1%) of variation in liability to schizophrenia is
captured by SNPs. We show that a substantial proportion of
this variation must be the result of common causal variants,
that the variance explained by each chromosome is linearly
related to its length (r = 0.89, P = 2.6 x 10−8), that the genetic
basis of schizophrenia is the same in males and females, and
that a disproportionate proportion of variation is attributable
to a set of 2,725 genes expressed in the central nervous system
(CNS; P = 7.6 x 10−8). These results are consistent with a
polygenic genetic architecture and imply more individual
SNP associations will be detected for this disease as sample
size increases.
The SNPs for SZ are apparently everywhere and nowhere. This is like trying to find out what subset of snowflakes is responsible for a blizzard. Heck, if we could do that, we could use Star Wars Missile Defense technology to zap those causal snowflakes out of the sky, and now no more snow days to keep docile kids from sitting in class all day having their minds buried by mediocre mass education. Note that the abstract interprets the findings as only supporting genetic Voodoo (or the existence of countless infinitesimal mischievous SNPs dancing in the head of a gene hunter), when, to the contrary, if (as stated in abstract) the distribution of the SNPs is across all chromosomes, and linearly related to chromosome length, this could be the signature of randomness or stochasticity – that is, noise. Noise in developmental genetic regulatory systems is inevitable, but it is not necessarily maladaptive. It can be captured and tuned by natural selection to create an optimized adaptive landscape of variation in any trait across a population. In fact, this is a universal strategy of life, and the genome can be thought of as an optimized “noise filter and exploiter”. The assertion that “a disproportionate proportion of variation is attributable to a set of 2,725 genes expressed in the central nervous system” might only tell us that every genetic locus involved in the development and function of a “normal” brain is involved in the SZ brain. Findings like these don’t answer etiologic questions. All they do is raise important, deep, urgent questions about what we’re studying in the first place. If the graded, nondiscrete (continuously distributed) behavioral syndrome of SZ is “caused” by prolific, nonsystematic, additive variation in the entire set of genes involved in CNS development and function, these findings might only be the signature of a quantitative, polygenic trait, like height, that is, the signature of variance about a monomorphic, canonical trait. Everybody has all the genes necessary for having “height”. No baby is born without them. From an evolutionary standpoint, the real question is what has fixed “height” as a universal (but variable) human trait, and what ecological or selective pressures might shape the distribution of variance around that trait across the population, resulting in a developmental gene regulatory architecture that guarantees diversity. As bipeds we require height in order to walk, to forage, to see above the grass, to free our hands to pluck fruit, throw spears, etc etc.
But variance in height, say within a mobile group of hunter gatherers, can be a valuable asset. It can provide an initial inequality in strength or power to accelerate the self-organization of reproductive dominance hierarchies and efficient division of labor with a cooperatively foraging/breeding/fighting group. A tunnel rat in Viet Nam was not the 6 foot 8 guy toting 100 pounds of ammo. He was a little guy who could also save the rest of his unit through his efforts, even when it turned into a suicide mission. The point-man was not a clumsy giant who would crash noisily through the bush, but a slender, lithe guy who could glide through the jungle like a whisper. And, tunnel rats and point men were usually low ranking, expendable. In baboons, the lowest ranking, most harassed males are more likely to forage at the periphery of the group, where they are more greatly exposed to the leopard. They may holler a shrieking alarm call right before they die. Thanks to the omega, the leopard and its cubs have been fed a delicious meal of low-ranking baboon meat, and the troop can sleep through the night under the fig tree. The long term evolutionary disadvantage of lack of variation around height (or just about any trait) is self evident. Natural selection “strives” to distribute trait-related risks and benefits across an “optimized” (not maximized) probabilistic landscape. Organisms tend to evolve probabilistic phenotypic distributions that roughly match the probability distributions of various situations in the environment of evolutionary adaptation (EEA, a Darwinian biological reality, not the Equal Environments Assumption, an exercise in Voodoo). In fact, evolution has no alternative. The abiotic world is inherently stochastic and never fully predictable. In social evolution, perfect phenotypic uniformity would always be trumped by an adaptive distribution of variance in species that live in subdivided metapopulations (e.g., mobile omnivorous hunter-gatherers) with a trickle of interbreeding, moving about in highly variable and unpredictable environments, and experiencing horrible stochasticity in colonization/extinction events. When one group encounters another at an oasis, what to do? Cooperate? Kill? These are evolutionary issues concerning universal laws governing the living state, and most of the gene hunters in human “behavioral genetics”, I will say with an intentional gust of rhetorical hyperbole, have never heard of Evolution. As Ken Weiss said in “Mr. Darwin’s misfortune: The burdens of knowing too much” (2011. Evolutionary Anthropology 20:43-47), “Many geneticists would not recognize a whole organism if they bumped into one in broad daylight.” I would say that many human behavioral geneticists wouldn’t recognize an evolved cooperatively breeding organism even if they looked in a mirror (although tremendous work on sociogenomics is being done in insects).
It so happens that on almost the same day that Charney’s commentary was posted on ISN the same group published a follow-up open-access article in the Am. J. of Human Genetics called “Additive Genetic Variation in Schizophrenia Risk Is Shared by Populations of African and European Descent”. The PDF can be obtained here:
http://www.sciencedirect.com/science/article/pii/S000292971300325X
Inspired by their “discovery” of myriad SNPs associated with SZ in Europeans, they sought to use the magic of GCTA to super-compare Africans and Europeans. They discovered that there was major overlap in the dire SNP/SZ profiles in African descent and European descent. This compelled them to proclaim that “many schizophrenia risk alleles are shared across ethnic groups and predate African-European divergence.” No problem with that. Alright so far! The genes (actually, a developmental gene regulatory system which is peppered with myriad neutral and near-neutral variants) for “height” also predated human exodus from Africa (by millions of years, in fact). But then they subjected their results to Festinger Phenomenization: they make it sound like they are moving closer to fully cataloguing the subset of snowflakes responsible for a very big human blizzard. But their findings actually support picture of “SZ” as an ancient, ubiquitous, and robust phenotypic potential in the human species that is there because of evolutionary processes, not because of getting toxoplasmosis from cat litter or because of not eating enough omega-3 fatty acids.
I suppose I should capitulate to the Voodoo, and imagine an enlightened future world of personalized genetic medicine in which there are 2,725(+) bad genes for SZ, and 2,725(+) medications to take. In fact, by profiling kids by amniocentesis, we can have a huge individualized cabinet full of preventive medicine awaiting each child, if we haven’t already performed a eugenic abortion.
When it comes to human “behavioral genetics”, the field overall has hardly made any progress since Darwin contemplated human nature. Many – not all, of course – behavioral geneticists are like chimps puzzling over a wristwatch or a bust of Freud. Can you use it to extract termites or crack a nut? Those who study irrationality are really studying themselves, not schizophrenics. Rationality is a graded phenotype. There are even a few nuclear physicists who believe in the Virgin Birth; there are national leaders who believe the earth was created 6,000 years ago; and there are gene hunters who can’t tell the SNP signature of evolution from a shoebox full of rocks. There are human biologists and medical scientists who do not realize that we are the alpha species on the Planet of the Apes.
Evan Charney is right. This is a doomed paradigm, but a paradigm that naively proclaims that it is “alright so far” or “getting ever closer to triumph”, like someone who has just walked through a lethal dose of radiation. Wait a little longer. However, we should be prepared for a predictable, desperate final stage of the paradigm failure, when some on the inside, those with the most to lose, recognize its doom and carry on fraudulently, like inside traders. Such fraudulent behavior would present as cooked data in a glossy EnRon prospectus or in a careful audit of EnRon’s books or internal memos. Could anyone do such a thing? There are great artists who have applied all of their gifts to producing forgeries. There are people with amazing scientific talents who produce forgeries. Rational deception, like irrational self-deception, is an evolved human “trait” too. When the resistance persists, even after overwhelming evidence against Voodoo, it might be something other than the Festinger Phenomenon.
The following reply is a response to M C Jones, September 22, 2013 at 7:15 pm.
Your comparison between scientists that endorse the GCTA approach to practitioners of voodoo is very tenuous. Your paranoid theory that a large segment of the scientific community is so devoted to the GCTA approach that they are completely unwilling to even question its validity is not even remotely supported by the paltry evidence you provide. If you really think some kind of ‘cognitive dissonance’ is compelling researchers to deliberately avoid the full range of situations that are required to adequately test GCTA, why not describe these glaring omissions? You mention what you feel is wrong with how the method was applied, but why not mention how it should have been used demonstrate its validity? This should be fairly easy for you considering you don’t share the fervor and devotion that supposedly afflicts those who endorse the GCTA approach.
The concepts that underlie mixed-effects models and their application to genetic markers is far from voodoo. In fact, they have proven their utility and validity in understanding heritable traits in livestock for over 3 decades (see Henderson’s Applications of Linear Models in Animal Breeding).
Your attitude regarding the application of GCTA to SNP data is reminiscent of how people’s attitudes toward technology are often conflated with magic. In many ways, especially for non-scientists, technological breakthroughs seem magical. For those who don’t know any better, believing in magic seems just as rational as believing in the “miracles” of science. For thousands of years humans have created “just so” stories to explain the unexplainable, such as your deductions based on hunter gatherer societies, and the effects of “reproductive dominance hierarchies,” but technology is not magic. There is a world of difference between your critiques based on speculative theorizing and the mathematically rigorous methods employed by GCTA in addition to most of approaches applied by population geneticists.
Also, many of the arguments you present in the section following “The SNPs for SZ are apparently everywhere and nowhere” suggest lack of familiarity with certain fundamental concepts of population genetics. This naiveté is also suggested by how much weight given to selection when you describe the factors that influence genetic variation within a population, rather than acknowledging the much more significant influences of genetic drift, and population bottlenecks that is supported by both theory and the results of marker based analysis. While there examples of selection signatures in the human genome, these sites represent only a very small fraction of all alleles at intermediate frequencies, and the observed distributions of alleles fit much better with Fisher’s infinite sites approximation rather than a mutation-selection balance model.
-Matt
I am very interested in your thinking about the adaptive value of human genetic diversity. Do you have any other writing along these lines?
I agree that (at least in the short term) our persistence in trying to understand the relationship between genome and organism is an example of the triumph of hope over experience. Because our genetic structure (and the associated biochemical mechanisms) are evolved, there is no pressure on them “make sense”. We’ll see how it shakes out, but the missing heritability is a hint that we’re going to find the structure of the genome is far more complex than we might have preferred, and may prove strongly resistant to reductive analysis.
In a somewhat oblique analogy, ideas of complexity and undecidability from computer science give some theoretical rigor to the idea that for sufficiently complex systems, the only way to see what the system is going to do is to watch it in action, and the only way to see the causal effect of a modification is to make that change and see what it does. If true, this doesn’t rule out the possibility of increased functional understanding and intentional manipulation of the genome, but it does mean that we would be heavily reliant on computer simulations and biological experiments, rather than a royal road of analytic insight.
@robamacl, http://humancond.org
Plomin is a eugenicist with ties to the pioneer fund. He has been chasing “the bell curve” theory of difference in intelligence between races for decades and most of his claims are simply bio-psycho-socialist speculation and surmise.
In 10000 B.C., there were a about 1 million of us, by 5000 B.C. there were 25 million, and until about five hundred years ago, the population was around 500 million. We are all descendants of just a small group of humans. All races can mate with each other. This tells us that we are all 99.9% genetically identical. So if we are so similar, how can we be so different? The eugenist claims never made sense once we figured this out.
The problem is that we were fooled by outward differences between us such as facial features, eye color, hair color and skin color and to a smaller extent, height. Hitler used such bigoted personal beliefs as justification to exterminate the more closely clustered jewish community. Our differences, however, are slight and like the variation of the colors on peacocks. But the genetic structure that develops the brain, kidneys, bones, etc. are exactly the same.
Heart kidney liver disease is a misnomer. It is damage. Schizophrenia, autism, low intelligence, cancer, allergies, immune disorders etc. these are all complex disorders caused by damage, especially during early childhood, not because of genetics. Why do you think that in the last 100 years, the rates of these diseases and disorders have increased exponentially? For 10000 years, we saw schizophrenia in one out of ten thousand and now we see it in one out of a hundred. Twenty to forty percent of the population now has ADHD or minimal brain damage. On the other hand, we put neurotoxins like lead in gas. We dumped our toxic waste into our rivers. You should not eat more than one serving of fish a week! We are now dumping millions of tons of toxins into our drinking water to extract methane. Most people living around chemical, paper, or coal power plants have various forms of cancer and ailments.
Good for you, Mr. Charney. We need more intelligent thought like this. The problem is our scientists are afraid to challenge the corporations that did this to our environment, it is easier to follow the herd mentality, and independent thought puts you in the cross hairs of the industry thug scientists which will attack you personally and not think twice to charge you in public. Ask Dr. Herbert Needleman what they did to him.
http://www.publichealthreports.org/issueopen.cfm?articleID=1479
Very poorly written article, especially when it was clearly written by someone who has a reasonable amount of background on the subject. The GCTA approach has actually validated the results of twin models and demonstrated that the assumption of equal environments was largely correct. The somewhat lower heritability estimates are exactly what is expected when COMMON SNPs in UNRELATED INDIVIDUALS are used to perform such analysis. The GCTA approach can actually be applied to samples of related individuals and you get the exact same estimates that are found using classic twin models.
I think the confusion in the article arises from misunderstanding how the method works at a fundamental level. The approach actually uses the same logic as twin studies, in that it determines how well you can predict phenotypic similarity from genetic similarity. In family studies, genetic similarity between subjects is estimated based on the fact that relatives share known proportions of their genomes. The reason GCTA works is because all “unrelated” people are in fact related to each other, albeit only distantly. So just like family based studies, GCTA determines how much of the genome is shared between seemingly unrelated individuals based on how many versions of SNP markers they have in common. SNP based similarity is then used to predict phenotype similarity, and you get an estimate of how much of the heritability for a given trait is captured by COMMON SNPs. This will only be the heritability captured by common SNPs when subjects are unrelated, and has the added benefit of no longer having to worry about the equal environments assumption because with the large sample size the law of large numbers will cause any variation in environment to cancel out. The reason this heritability estimate is less than an estimate generated from relatives (either using GCTA or classic family methods) is that rare-variants (markers too rare to be on a SNP platform) are not included in the estimate. Family based studies necessarily include rare-variant effects because relatives have the same probability of sharing a rare-SNP as they do a common-SNP. A concrete example comes from twins, where identical twins share all common and rare SNPs, and fraternal twins share half of their genomes (which includes both rare and common SNPs). The reason the GCTA approach will give you the EXACT SAME HERITABILITY ESTIMATE PROVIDED BY FAMILY STUDIES when applied to relatives is because even though you are only directly measuring genetic similarity between relatives using common SNPs, the probability of sharing common and rare SNPs within family members is perfectly correlated, like in the twin example above. So if GCTA shows sibling A shares half of his common SNPs with sibling B, this is necessarily the case for the rare SNPs they share as well. Because genetic recombination breaks up this correlation structure over generations, individuals who are seemingly unrelated no longer share the same rare-variants, and while they do share some distant common ancestors that imparted them with the same common SNPs, more recent relatives that they did not share gave them other variants that have not had time to reach common frequencies in the population.
The method is sensitive to population stratification, but these effects can be easily and robustly controlled for using estimates of the broad influences of population structure. I would explain how, but the articles are freely available through Nature Publishing and I’m sure you can all easily find them.
-Best, Matthew A. Simonson PhD
You are so lost in the trees that you have forgotten that there is a forest. Lets use intelligence as an example. Explain to me which genes are responsible for intelligence? Assuming you can name any, which no one has verifiably been able to, why are these genes responsible for intelligence? How many generations and chance changes does it take to make one more or less different for there to be a manifestation in a process based on a tiny difference?
Let me use an example. You are saying that a one hundred story skyscraper can be affected because a piece of irrelevant molding on story 40 is loose (Even this may be too big a variance to use as an example). Recently, the FDA has halted the use of companies making nonsensical genetics claims:
http://www.bloomberg.com/news/2013-11-25/fda-tells-google-backed-23andme-to-halt-dna-test-service.html
There is very very little difference (let me add a few more very to that) between us. The differences between us are so so so small that genes are not causative for anything. Skin color, eye color, hair color took thousands of years to change and involve just a few changes. Height, which involves several hundred to several thousand changes is only heritable at around 10%. A process, like intelligence, that involves millions upon millions of genes cannot be handed down from your parents or your clan. 50k years ago, there were a million of us.
We are learning that we can correct problems by tweaking things (unclogging the sink, restoring the corroded connection):
http://www.npr.org/2013/12/06/209618161/can-hacking-the-brain-make-you-healthier
If you can fix it by tweaking it, it was not built wrong – the environment made it that way. Until you start coherently explaining steps one (what), two (how), and three (why) – your claims belong in Star or Globe, not in scientific journals.
Response to Simonson
Let me be clear that my comment contains no misunderstanding as to how the GCTA method works “at a fundamental level.” Here was my characterization:
“Like a typical GWAS, GCTA involves scanning hundreds of thousands of polymorphisms (specifically, a common form of gene variant known as a single nucleotide polymorphism [SNP]) of thousands of persons. But instead of trying to identify individual polymorphisms more common among those who share a given trait, the goal is to determine whether or not this trait similarity can be associated with a large number of (unidentified) polymorphisms.”
I took it as a given that the reader would not assume that I was talking about thousands of related persons. So, GCTA scans the genomes of thousands of unrelated persons to determine if those who are concordant for a given “trait” e.g., height, intelligence, diabetes, etc., share more SNPs than those (unrelated individuals) who are not concordant for the trait. Yes, GCTA is based upon the assumption that we are all descended from a common ancestor (which of course, we are), a fact in no way relevant to my short exposition.
So perhaps Simonson thinks that my misunderstanding arises from the fact that GCTA is based upon common genetic variants, whereas twins will share both common and rare genetic variants. I am well aware of this. The reason why Simonson puts such an emphasis upon this is that he assumes that this explains the difference in heritability estimates derived from CGTA as opposed to twin studies:
“The somewhat lower heritability estimates are exactly what is expected when COMMON SNPs in UNRELATED INDIVIDUALS are used to perform such analysis. The GCTA approach can actually be applied to samples of related individuals and you get the exact same estimates that are found using classic twin models.”
This is simply not the case. Let us take a look at these “somewhat lower” heritability estimates. Consider the results published in a recent article, Maciej Trzaskowski, Philip S. Dale, and Robert Plomin (2013) “No Genetic Influence for Childhood Behavior Problems From DNA Analysis,” Journal of the American Academy of Child & Adolescent Psychiatry 52 (10)1048-1056.e3
Below, some of the authors’ heritability estimates based upon the twin study methodology (2,500 UK-“representative” 12-year-old twin pairs) are compared with their heritability estimates based upon GCTA (derived from 1 member of the twin pair with genotype data for 1.7 million DNA markers):
Twin methodology GCTA
MFQ depression: 40% 0
SDQ conduct: 38% 0
SDQ hyperactivity: 44% 0
SDQ peer: 40% 0
SDQ total: 42% 0
Connors hyperactivity: 80% 0
APSD psychopathic: 50% 0
MFQ depression: 73% 0
SDQ total: 60% 0
CAST autistic: 48% 0
Somewhat lower heritability estimates? The GCTA approach can actually be applied to samples of related individuals and you get the exact same estimates that are found using classic twin models?
And lest I be accused of only listing those results where the GCTA heritability estimate was 0, here are some comparisons in which the GCTA heritability estimate was not zero:
Twin methodology GCTA
APSD psychopathic 60% 16%
CAST autistic 72% 10%
Let me now turn to population stratification. According to Simonson, the effects of population “can be easily and robustly controlled for using estimates of the broad influences of population structure.”
This is not the case. Drawing on ideas largely developed in the field of single marker regressions, Yang et al. (2010) propose to account for population structure by adding to the whole-genome random regression (WGRR) model that fit all markers simultaneously, the dominating eigenvectors with coefficients treated as fixed effects.
The model could be of the form
y = μ+Σdi=1Uiαi+Wb+e
= μΣdi=1Uiαi+g+e,
where d is the number of the eigenvectors with the largest eigenvalues, often 10 or 20, and the α′s in Σdi=1 Uiαi are estimated by least squares (i.e. estimated without shrinkage). However this approach, suffers from “double counting” since the same eigenvectors whose coefficients are included as fixed effects enter, implicitly, as random effects in the random part of the model. The consequences on inferences of fitting this model are highly dependent on the distribution of marker genotypes in the data (see Janns et al. 2012).
We have found evidence of significant population stratification using the GCTA methodology on data from the Framingham Heart Study. We found that estimates for the heritability of the top hits from a GWAS using only those that graduated from college explained none of the heritability for those that did not attend college. The opposite version (heritability for those who attended college after removing top hits from a GWAS on those that did not attend college) was also true. This suggests that SNP effect estimates are extremely sensitive to environment.
The problem with all the “GCTA estimates are lower than twin study estimates” stuff that this article (and many comments on it) make such a big deal about is that they’re SUPPOSED to be lower, because GCTA estimates are LOWER BOUND estimates rather than best estimates of heritability. For instance, Plomin et al. (2013) note that “GCTA provides a lower-limit estimate of heritability because it misses genetic influence due to causal variants that are not highly correlated with the common SNPs on genotyping arrays.” https://www.ncbi.nlm.nih.gov/pmc/articles/PMC3652710/ More recently a similar point was made by Cheesman et al. (2017): “GCTA estimates of genetic influence, known as SNP heritability, will be lower than twin study-based heritability, partly because GCTA detects only the additive effects of causal variants tagged by common SNPs on current DNA arrays used in GWA research, and not non-additive effects or rare variants.” Thus arguing that lower GCTA estimates prove that twin study estimates are too high, as Charney does here, is simply false.
Sorry, forgot to include link to Cheesman et al. (2017). Here it is: https://www.ncbi.nlm.nih.gov/pmc/articles/PMC5802501/
I appreciate the detailed reply Evan, as well as the thorough use of citations employed when attempting to back up your position.
You mention “Yes, GCTA is based upon the assumption that we are all descended from a common ancestor (which of course, we are), a fact in no way relevant to my short exposition.” The fact that this is the fundamental reason why GCTA works makes it very relevant to your exposition, especially in the context of any comparison of the method with family based methods. Both methods are able to generate heritability estimates because they approximate how much of the genome is identical by descent (as in, descended from a COMMON ANCESTOR) between any two subjects, and then relate this to how similar their phenotype is. The cause of the differences in results obtained from the two methods, both in terms of being able to detect rare-variant influences, and the effects of shared environmental confounds, is a function of the degree of relatedness between subjects in the examined sample.
Also, I want to want to clarify why I emphasized that GCTA will produce very different results when applied to related vs. unrelated individuals. You mention that you “took it as a given that the reader would not assume that I was talking about thousands of related persons.” This is the very reason why I mentioned it so emphatically, your initial discussion of the method demonstrates that you were not aware that it can be applied to thousands of related subjects; see “Assumption-Free Estimation of Heritability from Genome-Wide Identity-by-Descent Sharing between Full Siblings”. While the software package GCTA didn’t exist at the time of this publication, Peter Visscher was applying the method used by GCTA to genotype data from thousands of sets of siblings to generate heritability estimates. This is summarized nicely in this quote: “Our application shows that it is feasible to estimate genetic variance solely from within-family segregation and provides an independent validation of previously untestable assumptions. Given sufficient data, our new paradigm will allow the estimation of genetic variation for disease susceptibility and quantitative traits that is free from confounding with non-genetic factors and will allow partitioning of genetic variation into additive and non-additive components.” I should also mention that the heritability estimate for height of 0.80 they obtained is effectively the same as those from classic twin methods.
You also state: “So perhaps Simonson thinks that my misunderstanding arises from the fact that GCTA is based upon common genetic variants, whereas twins will share both common and rare genetic variants. I am well aware of this. The reason why Simonson puts such an emphasis upon this is that he assumes that this explains the difference in heritability estimates derived from CGTA as opposed to twin studies:”
I should clarify that I definitely think a major contributor to the difference between twin studies and GCTA is the effect of rare-variants, but not the only factor. When you compare these results from GCTA with unrelated subjects to the results found in, “Assumption-Free Estimation of Heritability from Genome-Wide Identity-by-Descent Sharing between Full Siblings”, it’s fairly clear that rare-variants have an effect, but are not the entire story. A good summary of other potential sources of the differences in estimates from both methods comes for an article that you cited, “No Genetic Influence for Childhood Behavior Problems From DNA Analysis.”
In the first sentence of the discussion section the authors ask “Why do GCTA estimates show no significant genetic influence for diverse childhood behavior problems as rated by parents, teachers, or children themselves, even though twin study estimates of heritability are significant and substantial in the same sample using the same measures, and even though GCTA estimates for cognitive traits are significant and substantial?” (Hmm…, I don’t recall you mentioning this section in bold before?), they then go on to describe several potential explanations for the observed differences in detail, and while several potential explanations are given as alternatives to inaccuracy of twin models due to violation of the equal environments assumption, the authors finally concede: “In the absence of a more parsimonious explanation, we suggest that nonadditive genetic effects for behavior problems in childhood are masked by a general inflation of twin similarity for both MZ and DZ twins. One possibility is that this general twin inflation could be due to experiences that are shared by members of both MZ and DZ twin pairs.“
I found one section of the paper especially interesting and succinct when explaining the relationship between GCTA and and family based methods:
“As expected from the literature, the twin study heritability estimates for height and weight are about 80% and the estimates for the cognitive traits are about 50% (40%–60%). The GCTA estimates are about 40% for height and weight and about 25% (w20%–30%) for the cognitive traits. All of the GCTA estimates are statistically significant, as indicated by the standard errors. These significant and substantial GCTA estimates have 2 important implications. First, they validate the twin method. Second, they imply that sufficiently large GWA studies using current DNA arrays limited to additive effects of common SNPs should be able to account for about 50% of the heritability for height, weight, and cognitive traits. The finding that GCTA estimates are only one-half of the twin heritability estimates is similar to previous reports for these variables and could be due to several factors that either result in GCTA underestimates of twin heritability, such as nonadditive gene–gene interactions, gene–environment interactions, and rare alleles, or to factors that lead to inflation of heritability estimates in twin studies.“
I should mention that I was even more intrigued by the fact that you cited the article after having cherry-picked a couple sentences that supported you argument, even though the paper explicitly refutes one of the main assertions of your original essay. You claim “Thus far, GCTA studies appear to have proven critics of the twin study methodology right, yielding significantly lower heritability estimates… “, even when the results of the study we cite above, as well as the majority of results in the literature that have been produced by this method, generally disagree with your statement. In your response you conveniently focus on the results presented in figure 2, but why not also discuss figure 1?
Figure 1:
http://www.sciencedirect.com/science/article/pii/S0890856713005182#gr1
Figure 2:
http://ars.els-cdn.com/content/image/1-s2.0-S0890856713005182-gr2.jpg
When you attempt to refute the concordance between twin study estimates and GCTA based estimates of relatives “No Genetic Influence for Childhood Behavior Problems From DNA Analysis.” While this article fails to support the assertion you are making, I will get to that in a second.
cognitive traits but not for behavior problems. Third, the reason that our twin data do not indicate nonadditive genetic effects for behavior problems in childhood is that these nonadditive genetic effects are masked by inflated correlations for both MZ and DZ twins for ratings of behavior problems.”
I also want to point out that my original claim that:
“The somewhat lower heritability estimates are exactly what is expected when COMMON SNPs in UNRELATED INDIVIDUALS are used to perform such analysis. The GCTA approach can actually be applied to samples of related individuals and you get the exact same estimates that are found using classic twin models.”
THIS IS CORRECT, and your apparent attempt to refute it does not make sense. You state:
“This is simply not the case. Let us take a look at these ‘somewhat lower’ heritability estimates. Consider the results published in a recent article, Maciej Trzaskowski, Philip S. Dale, and Robert Plomin (2013) ‘No Genetic Influence for Childhood Behavior Problems From DNA Analysis,’ Journal of the American Academy of Child & Adolescent Psychiatry 52 (10)1048-1056.e3. Below, some of the authors’ heritability estimates based upon the twin study methodology (2,500 UK-“representative” 12-year-old twin pairs) are compared with their heritability estimates based upon GCTA (derived from 1 member of the twin pair with genotype data for 1.7 million DNA markers):
“
This study does not use GCTA to compare genetic similarity between relatives; it simply takes ONE individual from each pair of siblings and uses GCTA to determine heritability estimates (only including unrelated subjects). These estimates are then compared to the estimates generates using the classic twin methods. For a more accurate example of the GCTA approach applied to related subjects, see the first paper I mention above by Peter Visscher.
I stand by my assertion about the effects of population stratification as well, I recommend reading “Genome partitioning of genetic variation for complex traits using common SNPs,” by Yang et. al., specifically the section titled “ Quantifying the effect of population structure”
-Cheers, Matthew A. Simonson
I need to point out a typo that occurs right after I provide links to figures 1 and 2. This was accidentally pasted and should be ignored:
“When you attempt to refute the concordance between twin study estimates and GCTA based estimates of relatives “No Genetic Influence for Childhood Behavior Problems From DNA Analysis.” While this article fails to support the assertion you are making, I will get to that in a second.
cognitive traits but not for behavior problems. Third, the reason that our twin data do not indicate nonadditive genetic effects for behavior problems in childhood is that these nonadditive genetic effects are masked by inflated correlations for both MZ and DZ twins for ratings of behavior problems.”
I apologize for the numerous typos and sloppy writing, I was quickly writing my response while having breakfast, in addition to multitasking, I was writing before the effects of my morning cup of coffee had kicked in. Sloppiness aside, I stand by my arguments.
On re-reading this, there is one thing I want to clarify. In this section:
“even though the paper explicitly refutes one of the main assertions of your original essay. You claim “Thus far, GCTA studies appear to have proven critics of the twin study methodology right, yielding significantly lower heritability estimates… “, even when the results of the study we cite above, as well as the majority of results in the literature that have been produced by this method, generally disagree with your statement.”
When I say the paper does not agree with the critics of twin methodology, I mean that the differences in the estimates produced by GCTA vs. classic twin studies do not suggest that twin methods are invalid, just that the two methods are not measuring the same thing, and that the results of the observed results from both approaches appear to support each other when you consider GTCA is only estimating part of the narrow sense heritability, and twin studies are estimates of broad sense heritability.
-Matt
When you make your argument by criticizing another, you have lost the argument. On height, top down height is 60-80% heritable, but bottom up it is as low as 5%.
http://www.molecularecologist.com/2013/02/wheres-the-heritability-right-where-youd-expect-if-you-look-close-enough/
Why is there this missing heritability? Well some, like you, say it is because of missed rare variants that in combination have small effects which make them hard to detect without very large samples. Of course, the answer should be that very large samples should prove you right. But they won’t because in advanced society (last 2000 years) there has been massive integration and chance selection (slavery, travel, etc.) making such a claim quite silly:
http://www.councilforresponsiblegenetics.org/genewatch/GeneWatchPage.aspx?pageId=388
The problem is that when you get myopic about your views in this subject you start to cross lines – blaming the wrong thing because of a personal belief.
http://www.independent.co.uk/news/education/education-news/nature-nurture-and-education-michael-gove-and-the-question-of-genetics-in-schooling-8944579.html
The logical answer, however, is that your top down method fails to take into account the environment. The environment, such as toxic exposure or nutrition, play a far greater role (exponentially). The poor are poor because they are disabled. They are not disabled because of their genes. They are disabled and therefore poor. Poor people live in areas which have bad nutrition or are toxically exposed: An African community with bad nutrition, radioactive contamination (Chernobyl), a community residing around a coal plant or refinery, an older community where the walls have been painted with lead, or another community where the land is contaminated with pollutants (love canal) – these have far greater effects than the hypothetical and infinitesimally small slight variance genetics might potentially have.
http://www.ncbi.nlm.nih.gov/pubmed/12593879
The parents may have the same problems as their children – this does not mean it is genetic. This is the problem with top down. It fails to answer the what, why and how? You are making assumptions that have not and cannot be proven. Recently, they claimed the socioeconomic connection with intelligence was 2% based on 100,000 participants. This is a far cry from the 70% claimed by many neuropsychologists.
http://www.ncbi.nlm.nih.gov/pmc/articles/PMC3751588/
I am sure as that number increases to a million study participants, it will drop to .2%. As the sample population gets larger, genetic attribution will decrease incredibly to .1% or less. This is why you are chasing ghosts.
I want to begin by responding to the last comment of Simonson and then turn to a brief consideration of some of his substantive critiques, before turning to what I consider to be the heart of the matter, something that has not yet been touched upon in our exchanges.
Simonson’s last sentence is a response to my assertion that CGTA is particularly vulnerable to population stratification:
“I stand by my assertion about the effects of population stratification as well, I recommend reading “Genome partitioning of genetic variation for complex traits using common SNPs,” by Yang et. al., specifically the section titled “ Quantifying the effect of population structure”
I am not sure what to make of Simonson’s profession that he “stands by” his assertion in the absence of any argument. I have read Yang et al., and my technical comments concerning the vulnerability of GCTA to population stratification were directed specifically against that piece. As noted in my comment, population stratification is the number one reason why so many GWAS studies purporting to find particular SNPs associated with any number of traits have failed to be replicated.
It has taken scientists a long time to come to terms with the problem of population stratification in relation to the association between a SINGLE SNP and a well-defined physiological trait (and in fact, we still cannot adequately deal with it). Yang and Visscher believe that they have solved the problem (relying on formulas derived from animal breeding) for HUNDREDS OF THOUSANDS to MILLIONS OF SNPs. In the words of my colleague David Goldstein, Professor of Molecular Genetics & Microbiology and Professor of Biology and Director of the Center for Human Genome Variation at Duke University:
“The community learned how to control for artifact very well in considering single variant significance tests, but I do not think that we understand artifact in these experiments looking at genome wide estimates of heritability. For example, it is quite clear that subtle stratification not captured by taking the top few axes could inflate the estimates. I basically don’t buy it, but don’t think it is scientifically promising enough to even bother fighting. Now that said, unfortunately, it does influence how some people think about these traits. So it is kind of unfortunate in my view. Not very useful scientifically, leads to misunderstandings…” (correspondence on file with author).
So-called “cryptic relatedness” is omnipresent in all populations and it wreaks havoc with assumptions about “relatedness” and “unrelatedness” that cannot be “corrected for” by the statistical methods of Yang and Visscher. Consider, for example, the following recent study: Using data from 121 populations, Henn et al. [1] showed that the average amount of DNA shared IBD in most ethnolinguistically-defined populations, for example Native American groups, Finns, and Ashkenazi Jews, differs from continentally-defined populations by several orders of magnitude. Using extensive pedigree-based simulations, to predict degrees of relationship given the amount of genomic IBD sharing in both endogamous and ‘unrelated’ population samples, they identified tens of thousands of 2nd to 9th degree cousin pairs within a heterogeneous set of 5,000 Europeans.
Simonson comments:
“I should mention that I was even more intrigued by the fact that you cited the article after having cherry-picked a couple sentences that supported you argument, even though the paper explicitly refutes one of the main assertions of your original essay.”
The heritability estimates of GCTA in relation to the twin and family methodology are all over the map. I cited a number of other articles in my original comment where some heritability estimates were closer to twin study estimates (like Plomin’s article on intelligence) and other studies where it was minimal or zero. What we are witnessing is a whole range of ad hoc, conflicting explanations in an attempt to account for these discrepancies:
“Possible explanations for the remaining missing heritability are that the estimates of narrow sense heritability from twin and family studies are biased upwards, for example, by not properly accounting for nonadditive genetic factors and/or (common) environmental factors; rare variants that are not captured by common SNPs on current genotype platforms make a major contribution” [2]
“As mentioned earlier, GCTA under- estimates twin heritability because it captures only additive genetic effects tagged by the common SNPs used on GWA arrays. Gene–gene interactions, gene–environment interactions, and rare alleles will widen the gap between GCTA and twin estimates of heritability. However, it is not clear why this gap would be greater for behavior problems than for cognitive traits” [3].
“[Perhaps] twin studies overestimate heritability for behavior problems more than for cognitive traits. One reason to take this seriously is that twin studies yield higher estimates of heritability than do adoption studies for personality traits, which are related to behavior problems in that personality includes traits such as emotionality, impulsivity, and activity level” [3].
“The first hypothesis – that nonadditive genetic effects led to the low GCTA estimate of heritability for CU – is not supported by our twin results. As mentioned in the Methods section, the hallmark of nonadditive gene-gene (epistatic) interactions is that the DZ twin correlation is less than half the MZ twin correlation. However, in our twin analysis of CU, the DZ correlation (0.31) is almost exactly half the MZ correlation (0.63), providing no support for the hypothesis of nonadditive genetic influence” [4].
There is nothing like a “parsimonious explanation” here. What there is, is a significant discrepancy between the results of twin studies on the one hand and GCTA on the other.
Simonsen has misinterpreted my statement that it is not the case that heritability estimates derived from GCTA are “somewhat lower” than twin heritability estimates. GCTA estimates in general are frequently (not always) significantly lower than heritability estimates derived from twin studies, and frequently (not always) zero. It is a significant mischaracterization to describe the results of GCTA studies in general as yielding heritability estimates “slightly lower” than twin studies. That was the sum total of my point.
THE HEART OF THE MATTER
Now let me state that I believe that everything that I have just argued is in an important sense irrelevant, or if not irrelevant, then insignificant in relation to what the real problem is with GCTA. I have been arguing from within, as it were, the genetic paradigm that underlies the GCTA methodology by considering problems such as population stratification and the manner in which they undermine the validity of GCTA estimates. But there is something much more far reaching that undermines the validity of GCTA estimates, and that is the entire genetic paradigm on which they are based. For all of its presumed statistical sophistication, GCTA is based, FOUNDATIONALLY, upon a late 19th and early 20th century paradigm of “genes,” that in light of advances in molecular genetics over the past quarter century, has no scientific validity. Discussing how to reconcile the findings of twin studies with the findings of GCTA is like debating how to fit yet another planetary epicycle into the Copernican view of the solar system.
To defend this assertion adequately would require much more space than I have here (for a more extended discussion, I suggest my own “Behavior genetics and postgenomics” [5]). All that I will do here is list some fundamental scientific developments that do not, that CANNOT, coexist with the crude conception of genes and the genotype-phenotype relation that underlies the entire concept of heritability as expressed in twin studies, GCTA, behavior genetics, and all allied attempts to partition and quantify the effects of “genes” v. “environment” on phenotypic variation.
GCTA, like GWAS, uses blood samples or check swabs to identify and then scan “the human genome.” The problem with this is that persons do not have “a genome.” And I
am not even referring to mitochondrial DNA, which is inherited in a non-Mendelian manner and is simply ignored because it cannot fit into the simplistic, reductionist model upon which all heritability studies are based. What I am referring to is the fact that persons do not possess a single NUCLEAR genome. There is now overwhelming scientific evidence that the normal human condition is one of SOMATIC MOSAICISM, different DNA sequences (or “genomes”) in different cells and tissues of the body, a phenomenon that appears to be particularly prevalent in the human brain [6-18].
Conservative estimates place the overall percentage of aneuploid (a form of somatic mosaicism characterized by variable numbers of whole chromosomes – greater or less than 2 in a cell) neural cells in the normal adult brain at an astonishing 10%, involving monosomy, trisomy, polyploidy (greater than four chromosomes), and uniparental disomy (two copies of a chromosome from one parent [19 20]. Given an estimated 100 billion neurons in the adult brain, this yields a rough (conservative) estimate of 10 billion neurons and 100–500 billion glial cells (neural cells that do not transmit electrical impulses but play an essential role in neuronal structure and function) with one or another form of chromosomal aneuploidy. It is estimated that roughly 28% of embryonic neural precursor cells exhibit chromosomal aneuploidy in one form or another [14]. Mature aneuploid neurons are functionally active and integrated into brain circuitry, showing distant axonal connections. One likely result of this is neuronal signaling differences caused by altered gene expression, as documented in mammalian neural cells.
Suppose we are interested in the “heritability” of a psychological trait or “intelligence.” What, precisely, does the analysis of SNPs in DNA taken from blood or cheek cells tell us about the DNA in different regions of the brain, given widespread somatic mosaicism? (A further point is that because these processes are STOCHASTIC, MZ twins are discordant for DNA variation that results from somatic mosaicism [21]).
DNA is not the sole biological agent of inheritance. Persons inherit, in a non-Mendelian manner, epigenetic markings in the form of histone modifications and DNA methylation, non-coding RNAs including microRNAs and long non-coding RNAs, mitochondria and mitochondrial DNA, “maternal” oocytic messenger RNAs, and nucleoli (to name a few) [22-24] all of which play critical roles in every aspect of human development, phenotype formation, and phenotypic variation.
How are all of these non-DNA, non-Mendelian inherited elements to be incorporated into “gene-based” heritability estimates? The prevailing solution to this this problem is to ignore them. Furthermore, one cannot separate the “effect” of inherited DNA from the effect of all of the inherited elements just cited, because without these elements, DNA has no effect whatsoever (for more on this, see below).
With all but a few exceptions (so-called monogenic disorders and oligogenic disorders with a predisposing allele) the “presence” of a “gene,” whether one or one thousand, indicates very little about the “effect” of the “gene” because “gene effects” are the result of agents external to the gene, namely, the cell and all of the cellular machinery that turns genes on and off, transcribes genes, translates genes, and utilizes gene products. If a “gene” is epigenetically silenced, it cannot be transcribed, so the presence of the same “gene” in any two individuals does not tell us whether it can have any phenotypic effect. Furthermore, the presence of a given “gene” does not tell us what protein will be transcribed from it. There are estimated to be over 100,000 proteins in the human body – and the number may be significantly higher – yet approximately 25–30,000 genes in the human genome. Alternative splicing (AS) allows multiple transcripts to be produced from a single segment of DNA (“gene”) and, consequently, multiple proteins. AS is estimated to occur in 95% of human genes, and can result in numerous proteins being synthesized from the same “gene.” These “isoforms” can exert radically different and even opposed physiological effects [25-29].
The supposed dichotomy between “nature” and “nurture,” “genes” and “environment,” is an anachronism about as scientifically sound as Aristotle’s distinction between the sublunar world of change and the immutable heavens. Is the supposition supposed to be that everything “external” to the DNA sequence is “environment”? Is the chromatin, for example, in which the DNA is wrapped and which, by changing configuration in response to a variety of inputs, determines the extent to which any given segment of DNA is accessible to transcription factors and capable of being transcribed, part of the “environment”? Is the oocytic cytoplasm that contains “maternal” messenger RNAs that turn the zygotic genome on and that control early zygotic development prior to the activation of the embryonic genome, the “environment”?
Heritability studies assume that the DNA sequence (which DNA sequence?) does not change. As noted, DNA sequence changes during embryogenesis result in somatic mosaicism. What is more, retrotransposons or jumping genes, mobile segments of DNA that copy and paste themselves at various sites in the DNA sequence, changing DNA sequence and content, remain active throughout life in those parts of the brain that continue to generate new neurons (the hippocampus and the caudate nucleus) [30-35].
If behavior results from the accumulated activity of the embodied brain, if the DNA sequences vary in different neurons as a result of stochastic process during development, if the activity of the DNA sequences varies in different cells due to epigenetic differences, if these epigenetic differences vary during the developmental process and in different environments, if the DNA sequence continues to change in those parts of the brain that undergo neurogenesis throughout life as a result of the activity of retrotransposons, if the activity of these retrotransposons is itself regulated by the epigenome which in turn is highly responsive to environmental inputs, if the protein translated from a given segment of this DNA at any given time is determined not by the segment of the DNA itself but by the mechanisms of the cell which determine which isoform to transcribe in response to innumerable environmental outputs (and on and on), then how on earth are the common SNPs from DNA samples from blood cells of thousands of unrelated persons supposed to tell us, e.g., how much of the variation in depression, or “intelligence” (in a population) is “due to” “genes” and how much to “environment”?
HUMANS POSSESS FEWER GENES THAN CORN. One of the surprising findings of the Genome Project was that the human genome contains an estimated 20,000 protein coding “genes,” less than maize (i.e., corn), which contains over 32,000 protein-coding “genes” [36], and close in number to the nematode, with approximately 19,000. And many “genes” appear to be preserved across species. Surely, the distinctive properties of the human brain and human behavior are the result of something other than what we have less of than corn. Yet GCTA tells us that common SNPs, SNPs that we most likely share with corn and nematodes, account for 35% of the heritability of “intelligence.”
Finally, an explanation as to why I have put the words “gene” and “genes” in quotes throughout. Over one hundred years after the basic rules of heredity were established, the gene is undergoing an identity crisis. Indeed the question ‘‘what is a gene?’’ has been much debated in recent years [37-39]. I have already mentioned the fact that alternative splicing entails that potentially thousands of different proteins can be transcribed from the same gene. But what is this gene? Not clearly, a segment of DNA “coded” for the production of a particular protein. But “it” is likely not a segment of DNA at all. Proteins are produced by the use (by the cell) of various introns and exons, start cites and stop cites, and promoters that are by no means necessarily contiguous segments of DNA (as represented, for example, by an SNP) [40].
Practitioners of GCTA, twin studies, and behavioral genetics are either 1) completely unaware of advances in molecular genetics, 2) choose to ignore them because they cannot fit within their antiquated conception of genes and the genotype-phenotype relationship, or 3) believe that they are COMPATABLE with their underlying assumptions and methodologies. I am yet to see anything approaching a scientific defense of 3.
Let me attempt to forestall a common and misguided objection: that heritability estimates are in effect a form of biometrical analysis, whereas I have been focusing on “bio-molecular” genetics, something appropriately outside the purview of biometric genetics. The study of “heritability,” the objection goes, is concerned with the question, how much do two causal agents (“genes” and “environment”) contribute to phenotypic variation? Heritability is concerned with the question, how do various causal agents contribute to phenotypic variation (or phenotype in general)? This objection was always misguided, but in light of the growing pace of advances in molecular genetics and their far-reaching implications, it is particularly misguided today.
Almost every dogma that underlies heritability estimates has been upended by advances in molecular genetics: Persons do not possess “a genome;” the various “genomes” in the human body change over the course of development; the presence of a gene cannot be equated with its “effect,” i.e., production of a specific protein; and “genes,” particularly in the form of common SNPs do not distinguish humans from corn, so they certainly cannot be what distinguishes humans from each other.
1. Henn BM, Hon L, Macpherson JM, et al. Cryptic distant relatives are common in both isolated and cosmopolitan genetic samples. PloS one 2012;7(4):e34267-e67 doi: 10.1371/journal.pone.0034267[published Online First: Epub Date]|.
2. Vinkhuyzen AA, Pedersen NL, Yang J, et al. Common SNPs explain some of the variation in the personality dimensions of neuroticism and extraversion. Transl Psychiatry 2012;17(2):27
3. Trzaskowski M, Dale PS, Plomin R. No Genetic Influence for Childhood Behavior Problems From DNA Analysis. Journal of the American Academy of Child & Adolescent Psychiatry 2013;52(10):1048-56.e3 doi: http://dx.doi.org/10.1016/j.jaac.2013.07.016%5Bpublished Online First: Epub Date]|.
4. Viding E, Price TS, Jaffee SR, et al. Genetics of callous-unemotional behavior in children. PLoS One 2013;8(7):e65789 doi: 10.1371/journal.pone.0065789[published Online First: Epub Date]|.
5. Charney E. Behavior genetics and postgenomics. Behav Brain Sci 2012;35:1-80 doi: 10.1017/S0140525X11002226[published Online First: Epub Date]|.
6. Abyzov A, Mariani J, Palejev D, et al. Somatic copy number mosaicism in human skin revealed by induced pluripotent stem cells. Nature 2012;492(7429):438-42 doi: 10.1038/nature11629[published Online First: Epub Date]|.
7. Kano H, Godoy I, Courtney C, et al. L1 retrotransposition occurs mainly in embryogenesis and creates somatic mosaicism. Genes Dev 2009;23:1303-12
8. Baillie JK, Barnett MW, Upton KR, et al. Somatic retrotransposition alters the genetic landscape of the human brain. Nature 2011;479:534-37
9. Vitullo P, Sciamanna I, Baiocchi M, et al. LINE-1 retrotransposon copies are amplified during murine early embryo development. Molecular reproduction and development 2012;79(2):118-27 doi: 10.1002/mrd.22003[published Online First: Epub Date]|.
10. De S. Somatic mosaicism in healthy human tissues. Trends in genetics : TIG 2011;27(6):217-23 doi: 10.1016/j.tig.2011.03.002[published Online First: Epub Date]|.
11. Faulkner GJ. Retrotransposons: mobile and mutagenic from conception to death. FEBS letters 2011;585(11):1589-94 doi: 10.1016/j.febslet.2011.03.061[published Online First: Epub Date]|.
12. Frank SA. Somatic evolutionary genomics: Mutations during development cause highly variable genetic mosaicism with risk of cancer and neurodegeneration. PNAS 2009;107:1725-30 doi: 10.1073/pnas.0909343106[published Online First: Epub Date]|.
13. Iourov IY, Vorsanova SG, Liehr T, et al. Aneuploidy in the normal, Alzheimer’s disease and ataxia-telangiectasia brain: differential expression and pathological meaning. Neurobiology of disease 2009;34(2):212-20 doi: 10.1016/j.nbd.2009.01.003[published Online First: Epub Date]|.
14. Iourov IY, Vorsanova SG, Yurov YB. Detection of Aneuploidy in Neural Stem Cells of the Developing and Adult Human Brain. 2008;4(2):36-42
15. Martin SL. Jumping-gene roulette. Nature 2009;460(August)
16. Mkrtchyan H, Gross M, Hinreiner S, et al. Early embryonic chromosome instability results in stable mosaic pattern in human tissues. PloS one 2010;5(3):e9591-e91 doi: 10.1371/journal.pone.0009591[published Online First: Epub Date]|.
17. Muotri AR, Zhao C, Marchetto MCN, et al. Environmental influence on L1 retrotransposons in the adult hippocampus. Hippocampus 2009;19(10):1002-07 doi: 10.1002/hipo.20564[published Online First: Epub Date]|.
18. Sgaramella V, Astolfi PA. Somatic genome variations interact with environment , genome and epigenome in the determination of the phenotype : A paradigm shift in genomics ? 2010;9:470-73 doi: 10.1016/j.dnarep.2009.11.011[published Online First: Epub Date]|.
19. Rehen SK, Yung YC, McCreight MP, et al. Constitutional aneuploidy in the normal human brain. J Neurosci 2005;25(9):2176-80 doi: 10.1523/jneurosci.4560-04.2005[published Online First: Epub Date]|.
20. Iourov IY, Vorsanova SG, Yurov YB. Chromosomal variations in mammalian neuronal cells: known facts and attractive hypotheses. Int Rev Cytol 2006;249:143-91
21. Piotrowski A, Bruder CE, Andersson R, et al. Somatic mosaicism for copy number variation in differentiated human tissues. Hum Mutat 2008;29:1118-24
22. Charney E. Cytoplasmic inheritance redux. Adv Child Dev Behav 2013;44:225-55
23. Evsikov AV, Graber JH, Brockman JM, et al. Cracking the egg: molecular dynamics and evolutionary aspects of the transition from the fully grown oocyte to embryo. Genes & development 2006;20(19):2713-27 doi: 10.1101/gad.1471006[published Online First: Epub Date]|.
24. Schier AF. The maternal-zygotic transition: death and birth of RNAs. Science 2007;316(5823):406-7 doi: 10.1126/science.1140693[published Online First: Epub Date]|.
25. Grabowski P. Alternative splicing takes shape during neuronal development. Curr Opin Genet Dev 2011;21:388-94
26. Black DL. Mechanisms of alternative pre-messenger RNA splicing. Annu Rev Biochem 2003;72:291-336
27. Blekhman R, Marioni JC, Zumbo P, et al. Sex-specific and lineage-specific alternative splicing in primates. Genome Res 2010;20:180-89
28. Calarco JA, Xing Y, Caceres M, et al. Global analysis of alternative splicing differences between humans and chimpanzees. Gene Dev 2007;21:2963-75
29. Perriman RJ, Ares M. Alternative splicing variability: exactly how similar are two identical cells? Molecular systems biology 2011;7(505):505-05 doi: 10.1038/msb.2011.44[published Online First: Epub Date]|.
30. Perrat PN, DasGupta S, Wang J, et al. Transposition-driven genomic heterogeneity in the Drosophila brain. Science (New York, NY) 2013;340(6128):91-95 doi: 10.1126/science.1231965[published Online First: Epub Date]|.
31. Cowley M, Oakey RJ. Transposable elements re-wire and fine-tune the transcriptome. PLoS genetics 2013;9(1):e1003234-e34 doi: 10.1371/journal.pgen.1003234[published Online First: Epub Date]|.
32. Thomas Ca, Paquola ACM, Muotri AR. LINE-1 Retrotransposition in the Nervous System. Annual review of cell and developmental biology 2012;28:555-73 doi: 10.1146/annurev-cellbio-101011-155822[published Online First: Epub Date]|.
33. Iskow RC, McCabe MT, Mills RE, et al. Natural mutagenesis of human genomes by endogenous retrotransposons. Cell 2010;141(7):1253-61 doi: 10.1016/j.cell.2010.05.020[published Online First: Epub Date]|.
34. Muotri AR, Marchetto MCN, Coufal NG, et al. L1 retrotransposition in neurons is modulated by MeCP2. Nature 2010;468(7322):443-46 doi: 10.1038/nature09544[published Online First: Epub Date]|.
35. Muotri AR, Marchetto MCN, Zhao C, et al. Environmental influence on L1 retrotransposons in the adult hippocampus. Hippocampus 2009;19:1002-07 doi: 10.1002/hipo.20564[published Online First: Epub Date]|.
36. Schnable PS, Ware D, Fulton RS, et al. The B73 maize genome: complexity, diversity, and dynamics. Science 2009;326:1112-15
37. Mercer TR, Mattick JS. Understanding the regulatory and transcriptional complexity of the genome through structure. Genome Res 2013;23(7):1081-8 doi: 10.1101/gr.156612.113[published Online First: Epub Date]|.
38. Mudge JM, Frankish A, Harrow J. Functional transcriptomics in the post-ENCODE era. Genome Research 2013 doi: 10.1101/gr.161315.113[published Online First: Epub Date]|.
39. Brosius J. The fragmented gene. Ann N Y Acad Sci 2009;1178:186-93 doi: 10.1111/j.1749-6632.2009.05004.x[published Online First: Epub Date]|.
40. Mudge JM, Frankish A, Harrow J. Functional transcriptomics in the post-ENCODE era. Genome Res 2013;23(12):1961-73 doi: 10.1101/gr.161315.113[published Online First: Epub Date]|.
I want to begin by responding to the last comment of Simonson and then turn to a brief consideration of some of his substantive critiques, before turning to what I consider to be the heart of the matter, something that has not yet been touched upon in our exchanges.
Simonson’s last sentence is a response to my assertion that CGTA is particularly vulnerable to population stratification:
“I stand by my assertion about the effects of population stratification as well, I recommend reading “Genome partitioning of genetic variation for complex traits using common SNPs,” by Yang et. al., specifically the section titled “ Quantifying the effect of population structure”
I am not sure what to make of Simonson’s profession that he “stands by” his assertion in the absence of any argument. I have read Yang et al., and my technical comments concerning the vulnerability of GCTA to population stratification were directed specifically against that piece. As noted in my comment, population stratification is the number one reason why so many GWAS studies purporting to find particular SNPs associated with any number of traits have failed to be replicated.
It has taken scientists a long time to come to terms with the problem of population stratification in relation to the association between a SINGLE SNP and a well-defined physiological trait (and in fact, we still cannot adequately deal with it). Yang and Visscher believe that they have solved the problem (relying on formulas derived from animal breeding) for HUNDREDS OF THOUSANDS to MILLIONS OF SNPs. In the words of my colleague David Goldstein, Professor of Molecular Genetics & Microbiology and Professor of Biology and Director of the Center for Human Genome Variation at Duke University:
“The community learned how to control for artifact very well in considering single variant significance tests, but I do not think that we understand artifact in these experiments looking at genome wide estimates of heritability. For example, it is quite clear that subtle stratification not captured by taking the top few axes could inflate the estimates. I basically don’t buy it, but don’t think it is scientifically promising enough to even bother fighting. Now that said, unfortunately, it does influence how some people think about these traits. So it is kind of unfortunate in my view. Not very useful scientifically, leads to misunderstandings…” (correspondence on file with author).
So-called “cryptic relatedness” is omnipresent in all populations and it wreaks havoc with assumptions about “relatedness” and “unrelatedness” that cannot be “corrected for” by the statistical methods of Yang and Visscher. Consider, for example, the following recent study: Using data from 121 populations, Henn et al. [1] showed that the average amount of DNA shared IBD in most ethnolinguistically-defined populations, for example Native American groups, Finns, and Ashkenazi Jews, differs from continentally-defined populations by several orders of magnitude. Using extensive pedigree-based simulations, to predict degrees of relationship given the amount of genomic IBD sharing in both endogamous and ‘unrelated’ population samples, they identified tens of thousands of 2nd to 9th degree cousin pairs within a heterogeneous set of 5,000 Europeans.
Simonson comments:
“I should mention that I was even more intrigued by the fact that you cited the article after having cherry-picked a couple sentences that supported you argument, even though the paper explicitly refutes one of the main assertions of your original essay.”
The heritability estimates of GCTA in relation to the twin and family methodology are all over the map. I cited a number of other articles in my original comment where some heritability estimates were closer to twin study estimates (like Plomin’s article on intelligence) and other studies where it was minimal or zero. What we are witnessing is a whole range of ad hoc, conflicting explanations in an attempt to account for these discrepancies:
“Possible explanations for the remaining missing heritability are that the estimates of narrow sense heritability from twin and family studies are biased upwards, for example, by not properly accounting for nonadditive genetic factors and/or (common) environmental factors; rare variants that are not captured by common SNPs on current genotype platforms make a major contribution” [2]
“As mentioned earlier, GCTA under- estimates twin heritability because it captures only additive genetic effects tagged by the common SNPs used on GWA arrays. Gene–gene interactions, gene–environment interactions, and rare alleles will widen the gap between GCTA and twin estimates of heritability. However, it is not clear why this gap would be greater for behavior problems than for cognitive traits” [3].
“[Perhaps] twin studies overestimate heritability for behavior problems more than for cognitive traits. One reason to take this seriously is that twin studies yield higher estimates of heritability than do adoption studies for personality traits, which are related to behavior problems in that personality includes traits such as emotionality, impulsivity, and activity level” [3].
“The first hypothesis – that nonadditive genetic effects led to the low GCTA estimate of heritability for CU – is not supported by our twin results. As mentioned in the Methods section, the hallmark of nonadditive gene-gene (epistatic) interactions is that the DZ twin correlation is less than half the MZ twin correlation. However, in our twin analysis of CU, the DZ correlation (0.31) is almost exactly half the MZ correlation (0.63), providing no support for the hypothesis of nonadditive genetic influence” [4].
There is nothing like a “parsimonious explanation” here. What there is, is a significant discrepancy between the results of twin studies on the one hand and GCTA on the other.
Simonsen has misinterpreted my statement that it is not the case that heritability estimates derived from GCTA are “somewhat lower” than twin heritability estimates. GCTA estimates in general are frequently (not always) significantly lower than heritability estimates derived from twin studies, and frequently (not always) zero. It is a significant mischaracterization to describe the results of GCTA studies in general as yielding heritability estimates “slightly lower” than twin studies. That was the sum total of my point.
THE HEART OF THE MATTER
Now let me state that I believe that everything that I have just argued is in an important sense irrelevant, or if not irrelevant, then insignificant in relation to what the real problem is with GCTA. I have been arguing from within, as it were, the genetic paradigm that underlies the GCTA methodology by considering problems such as population stratification and the manner in which they undermine the validity of GCTA estimates. But there is something much more far reaching that undermines the validity of GCTA estimates, and that is the entire genetic paradigm on which they are based. For all of its presumed statistical sophistication, GCTA is based, FOUNDATIONALLY, upon a late 19th and early 20th century paradigm of “genes,” that in light of advances in molecular genetics over the past quarter century, has no scientific validity. Discussing how to reconcile the findings of twin studies with the findings of GCTA is like debating how to fit yet another planetary epicycle into the Copernican view of the solar system.
To defend this assertion adequately would require much more space than I have here (for a more extended discussion, I suggest my own “Behavior genetics and postgenomics” [5]). All that I will do here is list some fundamental scientific developments that do not, that CANNOT, coexist with the crude conception of genes and the genotype-phenotype relation that underlies the entire concept of heritability as expressed in twin studies, GCTA, behavior genetics, and all allied attempts to partition and quantify the effects of “genes” v. “environment” on phenotypic variation.
GCTA, like GWAS, uses blood samples or check swabs to identify and then scan “the human genome.” The problem with this is that persons do not have “a genome.” And I
am not even referring to mitochondrial DNA, which is inherited in a non-Mendelian manner and is simply ignored because it cannot fit into the simplistic, reductionist model upon which all heritability studies are based. What I am referring to is the fact that persons do not possess a single NUCLEAR genome. There is now overwhelming scientific evidence that the normal human condition is one of SOMATIC MOSAICISM, different DNA sequences (or “genomes”) in different cells and tissues of the body, a phenomenon that appears to be particularly prevalent in the human brain [6-18].
Conservative estimates place the overall percentage of aneuploid (a form of somatic mosaicism characterized by variable numbers of whole chromosomes – greater or less than 2 in a cell) neural cells in the normal adult brain at an astonishing 10%, involving monosomy, trisomy, polyploidy (greater than four chromosomes), and uniparental disomy (two copies of a chromosome from one parent [19 20]. Given an estimated 100 billion neurons in the adult brain, this yields a rough (conservative) estimate of 10 billion neurons and 100–500 billion glial cells (neural cells that do not transmit electrical impulses but play an essential role in neuronal structure and function) with one or another form of chromosomal aneuploidy. It is estimated that roughly 28% of embryonic neural precursor cells exhibit chromosomal aneuploidy in one form or another [14]. Mature aneuploid neurons are functionally active and integrated into brain circuitry, showing distant axonal connections. One likely result of this is neuronal signaling differences caused by altered gene expression, as documented in mammalian neural cells.
Suppose we are interested in the “heritability” of a psychological trait or “intelligence.” What, precisely, does the analysis of SNPs in DNA taken from blood or cheek cells tell us about the DNA in different regions of the brain, given widespread somatic mosaicism? (A further point is that because these processes are STOCHASTIC, MZ twins are discordant for DNA variation that results from somatic mosaicism [21]).
DNA is not the sole biological agent of inheritance. Persons inherit, in a non-Mendelian manner, epigenetic markings in the form of histone modifications and DNA methylation, non-coding RNAs including microRNAs and long non-coding RNAs, mitochondria and mitochondrial DNA, “maternal” oocytic messenger RNAs, and nucleoli (to name a few) [22-24] all of which play critical roles in every aspect of human development, phenotype formation, and phenotypic variation.
How are all of these non-DNA, non-Mendelian inherited elements to be incorporated into “gene-based” heritability estimates? The prevailing solution to this this problem is to ignore them. Furthermore, one cannot separate the “effect” of inherited DNA from the effect of all of the inherited elements just cited, because without these elements, DNA has no effect whatsoever (for more on this, see below).
With all but a few exceptions (so-called monogenic disorders and oligogenic disorders with a predisposing allele) the “presence” of a “gene,” whether one or one thousand, indicates very little about the “effect” of the “gene” because “gene effects” are the result of agents external to the gene, namely, the cell and all of the cellular machinery that turns genes on and off, transcribes genes, translates genes, and utilizes gene products. If a “gene” is epigenetically silenced, it cannot be transcribed, so the presence of the same “gene” in any two individuals does not tell us whether it can have any phenotypic effect. Furthermore, the presence of a given “gene” does not tell us what protein will be transcribed from it. There are estimated to be over 100,000 proteins in the human body – and the number may be significantly higher – yet approximately 25–30,000 genes in the human genome. Alternative splicing (AS) allows multiple transcripts to be produced from a single segment of DNA (“gene”) and, consequently, multiple proteins. AS is estimated to occur in 95% of human genes, and can result in numerous proteins being synthesized from the same “gene.” These “isoforms” can exert radically different and even opposed physiological effects [25-29].
The supposed dichotomy between “nature” and “nurture,” “genes” and “environment,” is an anachronism about as scientifically sound as Aristotle’s distinction between the sublunar world of change and the immutable heavens. Is the supposition supposed to be that everything “external” to the DNA sequence is “environment”? Is the chromatin, for example, in which the DNA is wrapped and which, by changing configuration in response to a variety of inputs, determines the extent to which any given segment of DNA is accessible to transcription factors and capable of being transcribed, part of the “environment”? Is the oocytic cytoplasm that contains “maternal” messenger RNAs that turn the zygotic genome on and that control early zygotic development prior to the activation of the embryonic genome, the “environment”?
Heritability studies assume that the DNA sequence (which DNA sequence?) does not change. As noted, DNA sequence changes during embryogenesis result in somatic mosaicism. What is more, retrotransposons or jumping genes, mobile segments of DNA that copy and paste themselves at various sites in the DNA sequence, changing DNA sequence and content, remain active throughout life in those parts of the brain that continue to generate new neurons (the hippocampus and the caudate nucleus) [30-35].
If behavior results from the accumulated activity of the embodied brain, if the DNA sequences vary in different neurons as a result of stochastic process during development, if the activity of the DNA sequences varies in different cells due to epigenetic differences, if these epigenetic differences vary during the developmental process and in different environments, if the DNA sequence continues to change in those parts of the brain that undergo neurogenesis throughout life as a result of the activity of retrotransposons, if the activity of these retrotransposons is itself regulated by the epigenome which in turn is highly responsive to environmental inputs, if the protein translated from a given segment of this DNA at any given time is determined not by the segment of the DNA itself but by the mechanisms of the cell which determine which isoform to transcribe in response to innumerable environmental outputs (and on and on), then how on earth are the common SNPs from DNA samples from blood cells of thousands of unrelated persons supposed to tell us, e.g., how much of the variation in depression, or “intelligence” (in a population) is “due to” “genes” and how much to “environment”?
HUMANS POSSESS FEWER GENES THAN CORN. One of the surprising findings of the Genome Project was that the human genome contains an estimated 20,000 protein coding “genes,” less than maize (i.e., corn), which contains over 32,000 protein-coding “genes” [36], and close in number to the nematode, with approximately 19,000. And many “genes” appear to be preserved across species. Surely, the distinctive properties of the human brain and human behavior are the result of something other than what we have less of than corn. Yet GCTA tells us that common SNPs, SNPs that we most likely share with corn and nematodes, account for 35% of the heritability of “intelligence.”
Finally, an explanation as to why I have put the words “gene” and “genes” in quotes throughout. Over one hundred years after the basic rules of heredity were established, the gene is undergoing an identity crisis. Indeed the question ‘‘what is a gene?’’ has been much debated in recent years [37-39]. I have already mentioned the fact that alternative splicing entails that potentially thousands of different proteins can be transcribed from the same gene. But what is this gene? Not clearly, a segment of DNA “coded” for the production of a particular protein. But “it” is likely not a segment of DNA at all. Proteins are produced by the use (by the cell) of various introns and exons, start cites and stop cites, and promoters that are by no means necessarily contiguous segments of DNA (as represented, for example, by an SNP) [40].
Practitioners of GCTA, twin studies, and behavioral genetics are either 1) completely unaware of advances in molecular genetics, 2) choose to ignore them because they cannot fit within their antiquated conception of genes and the genotype-phenotype relationship, or 3) believe that they are COMPATABLE with their underlying assumptions and methodologies. I am yet to see anything approaching a scientific defense of 3.
Let me attempt to forestall a common and misguided objection: that heritability estimates are in effect a form of biometrical analysis, whereas I have been focusing on “bio-molecular” genetics, something appropriately outside the purview of biometric genetics. The study of “heritability,” the objection goes, is concerned with the question, how much do two causal agents (“genes” and “environment”) contribute to phenotypic variation? Heritability is concerned with the question, how do various causal agents contribute to phenotypic variation (or phenotype in general)? This objection was always misguided, but in light of the growing pace of advances in molecular genetics and their far-reaching implications, it is particularly misguided today.
Almost every dogma that underlies heritability estimates has been upended by advances in molecular genetics: Persons do not possess “a genome;” the various “genomes” in the human body change over the course of development; the presence of a gene cannot be equated with its “effect,” i.e., production of a specific protein; and “genes,” particularly in the form of common SNPs do not distinguish humans from corn, so they certainly cannot be what distinguishes humans from each other.
1. Henn BM, Hon L, Macpherson JM, et al. Cryptic distant relatives are common in both isolated and cosmopolitan genetic samples. PloS one 2012;7(4):e34267-e67 doi: 10.1371/journal.pone.0034267[published Online First: Epub Date]|.
2. Vinkhuyzen AA, Pedersen NL, Yang J, et al. Common SNPs explain some of the variation in the personality dimensions of neuroticism and extraversion. Transl Psychiatry 2012;17(2):27
3. Trzaskowski M, Dale PS, Plomin R. No Genetic Influence for Childhood Behavior Problems From DNA Analysis. Journal of the American Academy of Child & Adolescent Psychiatry 2013;52(10):1048-56.e3 doi: http://dx.doi.org/10.1016/j.jaac.2013.07.016%5Bpublished Online First: Epub Date]|.
4. Viding E, Price TS, Jaffee SR, et al. Genetics of callous-unemotional behavior in children. PLoS One 2013;8(7):e65789 doi: 10.1371/journal.pone.0065789[published Online First: Epub Date]|.
5. Charney E. Behavior genetics and postgenomics. Behav Brain Sci 2012;35:1-80 doi: 10.1017/S0140525X11002226[published Online First: Epub Date]|.
6. Abyzov A, Mariani J, Palejev D, et al. Somatic copy number mosaicism in human skin revealed by induced pluripotent stem cells. Nature 2012;492(7429):438-42 doi: 10.1038/nature11629[published Online First: Epub Date]|.
7. Kano H, Godoy I, Courtney C, et al. L1 retrotransposition occurs mainly in embryogenesis and creates somatic mosaicism. Genes Dev 2009;23:1303-12
8. Baillie JK, Barnett MW, Upton KR, et al. Somatic retrotransposition alters the genetic landscape of the human brain. Nature 2011;479:534-37
9. Vitullo P, Sciamanna I, Baiocchi M, et al. LINE-1 retrotransposon copies are amplified during murine early embryo development. Molecular reproduction and development 2012;79(2):118-27 doi: 10.1002/mrd.22003[published Online First: Epub Date]|.
10. De S. Somatic mosaicism in healthy human tissues. Trends in genetics : TIG 2011;27(6):217-23 doi: 10.1016/j.tig.2011.03.002[published Online First: Epub Date]|.
11. Faulkner GJ. Retrotransposons: mobile and mutagenic from conception to death. FEBS letters 2011;585(11):1589-94 doi: 10.1016/j.febslet.2011.03.061[published Online First: Epub Date]|.
12. Frank SA. Somatic evolutionary genomics: Mutations during development cause highly variable genetic mosaicism with risk of cancer and neurodegeneration. PNAS 2009;107:1725-30 doi: 10.1073/pnas.0909343106[published Online First: Epub Date]|.
13. Iourov IY, Vorsanova SG, Liehr T, et al. Aneuploidy in the normal, Alzheimer’s disease and ataxia-telangiectasia brain: differential expression and pathological meaning. Neurobiology of disease 2009;34(2):212-20 doi: 10.1016/j.nbd.2009.01.003[published Online First: Epub Date]|.
14. Iourov IY, Vorsanova SG, Yurov YB. Detection of Aneuploidy in Neural Stem Cells of the Developing and Adult Human Brain. 2008;4(2):36-42
15. Martin SL. Jumping-gene roulette. Nature 2009;460(August)
16. Mkrtchyan H, Gross M, Hinreiner S, et al. Early embryonic chromosome instability results in stable mosaic pattern in human tissues. PloS one 2010;5(3):e9591-e91 doi: 10.1371/journal.pone.0009591[published Online First: Epub Date]|.
17. Muotri AR, Zhao C, Marchetto MCN, et al. Environmental influence on L1 retrotransposons in the adult hippocampus. Hippocampus 2009;19(10):1002-07 doi: 10.1002/hipo.20564[published Online First: Epub Date]|.
18. Sgaramella V, Astolfi PA. Somatic genome variations interact with environment , genome and epigenome in the determination of the phenotype : A paradigm shift in genomics ? 2010;9:470-73 doi: 10.1016/j.dnarep.2009.11.011[published Online First: Epub Date]|.
19. Rehen SK, Yung YC, McCreight MP, et al. Constitutional aneuploidy in the normal human brain. J Neurosci 2005;25(9):2176-80 doi: 10.1523/jneurosci.4560-04.2005[published Online First: Epub Date]|.
20. Iourov IY, Vorsanova SG, Yurov YB. Chromosomal variations in mammalian neuronal cells: known facts and attractive hypotheses. Int Rev Cytol 2006;249:143-91
21. Piotrowski A, Bruder CE, Andersson R, et al. Somatic mosaicism for copy number variation in differentiated human tissues. Hum Mutat 2008;29:1118-24
22. Charney E. Cytoplasmic inheritance redux. Adv Child Dev Behav 2013;44:225-55
23. Evsikov AV, Graber JH, Brockman JM, et al. Cracking the egg: molecular dynamics and evolutionary aspects of the transition from the fully grown oocyte to embryo. Genes & development 2006;20(19):2713-27 doi: 10.1101/gad.1471006[published Online First: Epub Date]|.
24. Schier AF. The maternal-zygotic transition: death and birth of RNAs. Science 2007;316(5823):406-7 doi: 10.1126/science.1140693[published Online First: Epub Date]|.
25. Grabowski P. Alternative splicing takes shape during neuronal development. Curr Opin Genet Dev 2011;21:388-94
26. Black DL. Mechanisms of alternative pre-messenger RNA splicing. Annu Rev Biochem 2003;72:291-336
27. Blekhman R, Marioni JC, Zumbo P, et al. Sex-specific and lineage-specific alternative splicing in primates. Genome Res 2010;20:180-89
28. Calarco JA, Xing Y, Caceres M, et al. Global analysis of alternative splicing differences between humans and chimpanzees. Gene Dev 2007;21:2963-75
29. Perriman RJ, Ares M. Alternative splicing variability: exactly how similar are two identical cells? Molecular systems biology 2011;7(505):505-05 doi: 10.1038/msb.2011.44[published Online First: Epub Date]|.
30. Perrat PN, DasGupta S, Wang J, et al. Transposition-driven genomic heterogeneity in the Drosophila brain. Science (New York, NY) 2013;340(6128):91-95 doi: 10.1126/science.1231965[published Online First: Epub Date]|.
31. Cowley M, Oakey RJ. Transposable elements re-wire and fine-tune the transcriptome. PLoS genetics 2013;9(1):e1003234-e34 doi: 10.1371/journal.pgen.1003234[published Online First: Epub Date]|.
32. Thomas Ca, Paquola ACM, Muotri AR. LINE-1 Retrotransposition in the Nervous System. Annual review of cell and developmental biology 2012;28:555-73 doi: 10.1146/annurev-cellbio-101011-155822[published Online First: Epub Date]|.
33. Iskow RC, McCabe MT, Mills RE, et al. Natural mutagenesis of human genomes by endogenous retrotransposons. Cell 2010;141(7):1253-61 doi: 10.1016/j.cell.2010.05.020[published Online First: Epub Date]|.
34. Muotri AR, Marchetto MCN, Coufal NG, et al. L1 retrotransposition in neurons is modulated by MeCP2. Nature 2010;468(7322):443-46 doi: 10.1038/nature09544[published Online First: Epub Date]|.
35. Muotri AR, Marchetto MCN, Zhao C, et al. Environmental influence on L1 retrotransposons in the adult hippocampus. Hippocampus 2009;19:1002-07 doi: 10.1002/hipo.20564[published Online First: Epub Date]|.
36. Schnable PS, Ware D, Fulton RS, et al. The B73 maize genome: complexity, diversity, and dynamics. Science 2009;326:1112-15
37. Mercer TR, Mattick JS. Understanding the regulatory and transcriptional complexity of the genome through structure. Genome Res 2013;23(7):1081-8 doi: 10.1101/gr.156612.113[published Online First: Epub Date]|.
38. Mudge JM, Frankish A, Harrow J. Functional transcriptomics in the post-ENCODE era. Genome Research 2013 doi: 10.1101/gr.161315.113[published Online First: Epub Date]|.
39. Brosius J. The fragmented gene. Ann N Y Acad Sci 2009;1178:186-93 doi: 10.1111/j.1749-6632.2009.05004.x[published Online First: Epub Date]|.
40. Mudge JM, Frankish A, Harrow J. Functional transcriptomics in the post-ENCODE era. Genome Res 2013;23(12):1961-73 doi: 10.1101/gr.161315.113[published Online First: Epub Date]|.
– GCTA does not assume that SNPs cause the trait, only that they are linked with the causal genetic material. This could even be cross-generation-heritable epigenetic annotations.
– GCTA does not assume any sort of atomic gene concept, only that individuals share inherited stretches of DNA. It is true that the realities of DNA turned out to be more complex than the structure inferred by classic genetics. What “gene” means is now rather up in the air, but this doesn’t in any way undermine the idea that inherited genetic information explains the great similarity between all humans and the greater similarity of closely related humans. There is an emerging consensus that changes in non-coding DNA are extremely important to the differences between humans, other primates, and plants. Is this a “gene” or not? Dunno.
– Do epigenetics, mosaicism, and so on free humans from the tyranny of genetic determinism? They do mean the picture is more complicated than one might have supposed, but all along we knew that organisms adapt to their environments, in part by regulating gene expression. Insofar as these are adaptive mechanisms, they are mechanisms of gene regulation. They are keyed off of non-coding DNA, mediated by proteins coded by other DNA, functional RNAs expressed from DNA. It’s true that it’s a huge mess, and will resist understanding, but that doesn’t mean we should give up.
– Identical twins are conspicuously similar to each other in many ways, more similar than ordinary siblings or “unrelated” people. Twin studies attempt to quantify this, and in doing so make use of assumptions of varying plausibility. The conclusion from these studies of low gene x gene interaction (epistasis) is particularly puzzing, because at the micro scale biochemical processes are strongly interacting dynamic systems, and even classical genetics has non-additive dominance.
– Genome technologies will continue to cast light on the mechanisms by which our heritage underlies human diversity. GCTA is certainly not the last word, but of the current technologies seems to be the most relevant comparison to classical heritability techniques. Important next steps are using full sequence data to unpick the assumptions of linkage between SNPs and unknown nearby causal DNA.
@robamacl, http://humancond.org
Are the twin studies wrong? Well, no but they are subject to being misinterpreted. Down Syndrome (1-750) and Klinefelter Syndrome (1 in 500-1,000 live born males) are the most common genetic syndromes. Neither is inherited, they are caused by reproductive errors (sperm or egg). The MZ concordance rate for both these syndrome is near 100% while in DZ twins the concordance rate is near 0% giving these syndromes the highest ‘heritability’ estimates based on classic twin study design.
The rapidly advancing technology are finding that all males generate sperm mutations. The classic twin study can never reflect de novo genetic variances therefore the twin studies are certainly inflated.
Secondly, classical twin studies need to segregate MZ concordance rates by chorion type, monochorianic who share the same prenatal environment vs dichorionic (seperate placentas) who do not share exactly the same prenatal environment,
A CORRECTION!
In the second to last paragraph, I wrote:
The study of “heritability,” the objection goes, is concerned with the question, how much do two causal agents (“genes” and “environment”) contribute to phenotypic variation? Heritability is concerned with the question, how do various causal agents contribute to phenotypic variation (or phenotype in general)?
What I meant to write was:
The study of “heritability,” the objection goes, is concerned with the question, how much do two causal agents (“genes” and “environment”) contribute to phenotypic variation? BIO-MOLECULAR GENETICS is concerned with the question, how do various causal agents contribute to phenotypic variation (or phenotype in general)?
Thanks for the clarification of your opinions Evan. After reading your most recent post, I was curios about your research and decided to read some of your more recent papers. Given the fastidiousness of the counterclaims you provide in our discussion above, it makes a lot of sense that many of your publications espouse the theories you recapitulate above. I guess we will have to see if your arguments hold up in the long run; hopefully you really are ahead of the curve, and most mainstream geneticists just haven’t caught up yet.
-Matt
Great article Dr. Charney!
These days the only two groups of people who don’t believe in natural selection are religious fundamentalists and geneticists. I’m not sure which group spreads more harmful misinformation.
Since I was a teenager every disease has been studied as if it was caused by heredity. It’s so dumb.
Disease is caused by damage, not heredity. Most of that damage is in some way related to harmful inflammation. The world is exactly that simple but you’d never know that if you talked to someone involved in genetics.
There are truly good ideas here. But…
Don’t you think your criticism against classical twin studies is a little bit over-stated ? First, the simple fact that GWAS estimates is (much) lower than twin studies does not prove that the past criticisms were right. You should already know this; behavioral geneticists usually say that the study of rarer DNA variants should produce higher heritability estimates and that the current ones should be seen as lower-bounds. Furthermore, if a simple additive model predicts full sibling correlation to be half that of MZT, I believe the data supports the prediction, no ? And I also believe EEA certainly holds. Plus, I’m convinced that the common critics, among them were the chorion effects and environmental similarity, don’t hold water.
Derks Eske M., Dolan Conor V., Boomsma Dorret I. (2006). A Test of the Equal Environment Assumption (EEA) in Multivariate Twin Studies.
Loehlin John C. (1989). Partitioning Environmental and Genetic Contributions to Behavioral Development.
Bouchard Jr. Thomas J. (1983). Do Environmental Similarities Explain the Similarity in Intelligence of Identical Twins Reared Apart?.
Jacobs et al. (2001). Heritability Estimates of Intelligence in Twins: Effect of Chorion Type.
If there exists some serious flaws on twin studies, I’m still waiting for them. The empirical proofs don’t support it. Now, regarding what you say here :
“population stratification is the number one reason why so many GWAS studies purporting to find particular SNPs associated with any number of traits have failed to be replicated”.
I don’t follow the argument. It seems self-defeating to me. You criticize GWAS for over-estimating heritability but the above comment sounds as if you say it will reduce the accuracy of heritability estimates, i.e., its true estimates. Say it otherwise, more accurate estimations leads to higher heritability, not lower. It seems to me quite possible. Some researchers believe it :
Luciano, et al., (2013). A genome-wide association study for reading and language abilities in two population cohorts.
Previously implicated SNPs/genes associated with reading or SLI showed somewhat inconsistent, albeit potentially informative, results across our two cohorts. As previously reported by Luciano et al . (2007), rs2143340 in KIAA0314 was associated with word reading in BATS but was only marginally significant in ALSPAC. In a subsample of ALSPAC, which excluded non-white, very low IQ and potentially autistic children this SNP was, however, found to be significant (Scerri et al . 2011). Our finding suggests, then, that background population structure as discussed by Paracchini et al . (2008) can be a barrier to replication for genetic association.
Some other researchers are also skeptical that population stratification is a strong bias factor. See for example :
Davies G., et al., (2011). Genome-wide association studies establish that human intelligence is highly heritable and polygenic.
Can the results reported here be explained by population stratification or a correlation between environmental and genetic similarity? A number of reasons suggest strongly that these explanations are unlikely. The results were consistent when we estimated genetic variance within sub-populations and when we adjusted for up to 20 principal components (Supplementary Table 2). The observation that individual cohorts do not show an inflation of the test statistic, but the combined sample does, would require undetected spurious phenotype–genotype associations due to stratification in all cohorts to be in the same direction, which seems very unlikely. We recently showed that when investigating a trait under polygenic inheritance, increasing the sample size would indeed be expected to increase the inflation factor. A correlation between environmental and genetic similarity might occur if similarity due to environmental factors between relatives segregates with the degree of separation. For example, cousins five times removed might be more similar than cousins six times removed because they have a more similar environment. This argument applies to single SNP associations with any complex trait, and there is no evidence that the robustly associated variants from GWAS are spurious in this respect. Moreover, we estimated the actual amount of genome sharing between very distant relatives, which is different from the expected amount of sharing if we knew the entire pedigree of all individuals. In fact, the more distantly related a pair of individuals is from the pedigree, the larger the amount of variation in actual genome-wide sharing around this expectation (see Supplementary Information for further detail). Finally, we partitioned genetic variation to individual chromosomes by fitting the relationship matrices from all autosomes simultaneously in the model. For very distant relatives, as we have in our study, this method is robust to stratification.
“Almost every dogma that underlies heritability estimates has been upended by advances in molecular genetics: Persons do not possess “a genome;” the various “genomes” in the human body change over the course of development; the presence of a gene cannot be equated with its “effect,” i.e., production of a specific protein; and “genes,” particularly in the form of common SNPs do not distinguish humans from corn, so they certainly cannot be what distinguishes humans from each other.”
Except that humans do resemble one another, and more closely related individuals more so – an extreme example being monozygotic twins – MZ discordance is where all these alternative mechanisms will be leaving their fingerprints, if they are as important as you posit; the genes of biometrical genetics are not protein coding, they are phenotype altering variations, whether a single nucleotide changes in “intergenic” regulatory regions, small or large deletions, inversions or duplications; that development generally is tightly constrained, and those constraints are genetic programs; that somatic mutation will generally act to diminish germline genomic determination of the end phenotype; and that these approaches you so disparage are perfectly accepted for every organ, and for every interspecies difference, except in the human brain. In fact, behaviour geneticts love putting epistasis, epigenetics, tissue-specific gene regulation, gene-environment interaction, feedback loops between behaviours of interacting individuals, gene-environment covariation, etc etc in their grants, and if they detect anything, in their papers.
I knew there was some nonsense going on with these Twin and GWAS studies.
Thanks for posting this.
It made me laugh when I read that supplemental page. Especially when the same variant is associated with the opposite construct. Lol.
Best of luck.
I’m so glad this was posted, because it will (hopefully) stay online to ridicule the original poster and all his followers, who were so sure that GWAS is not the answer and will eventually turn out as a waste of time and money. Of course, this was largely based on their gut feeling and mixed with usual amount of pseudoscientific referencing. Although brave people like Matthew Simonson try to explain the basics of science, it is often better to let people embarrass themselves.
Evan Charney, September 2013:
“And to date, not a single polymorphism has been reliably associated with any psychiatric disorders nor any aspect of human behavior within the “normal” range (e.g., differences in “intelligence”).”
Nature, July 2014:
http://www.nature.com/nature/journal/v511/n7510/full/nature13595.html
I never knew the Eye color variants were only highly correlated and are predictive. They actually don’t know weather the alleles cause the difference. Same alleles can have opposite effects.
Is there any proof of what these alleles do other than just sit there? Its like that with most monogenic diseases too it seems. Even the rare mutations only predict an outcome.
This can also be seen in the famous MAOA Vntr. The low activity of the alleles are just an association. Low activity allele eg: MAOA 2R does not mean the gene will be low activity.
Here: No difference in MAOA activity in the brain and VNTR, but did associate with methylation:
http://www.ncbi.nlm.nih.gov/pubmed/22948232
… and here opposite activity in MAOA.
http://fsjournal.cpu.edu.tw/content/vol6.no.2/960202.pdf
I have a feeling some careers are going to be ruined soon.
Omg, even the lactose tolerance/intolerance gene variants are only correlations. Literally people with the same lactose variants can have opposite effects in the gene. The gene itself switches off in most people after weaning(sometimes before) and causing intolerance or tolerance if it stays on. So the variants can obviously do the same thing regardless of the variant, which you don’t need to have in order to have it switch on or off. These variants are just good for making a prediction but there is no proof that its the difference in variant that is causing the difference in gene expression. There is proof however that any variant can have the same or opposite effects.
The only gene variant I can find that is causative so far are the sickle cell causing ones. However these genes produce abnormal versions of what the rest of the variants do. Also you can actually detect the difference, its measurable. The other variants don’t seem have an effect difference between them.
Honestly I never knew the rabbit hole went this deep.
Good luck Evan Charney.